I recently exposed the Diversity Indices to all users on Microbiome Prescription. It is on the [Research Features] tab.
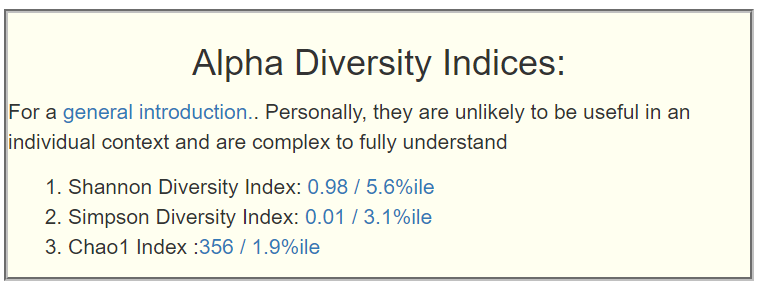
I then computed the average and also the average percentile for each symptom. I prefer to use percentile because the distribution is not a bell curve. Converting to percentiles transform it to a uniform distribution which is easier to work with for statistical tests. Percentiles are also easier for people to understand.
See this write up by CosmosId to explain these indices. I also attach the data as a CSV file for those who want to play with sorting the data in different manners. For all of the Rank, 50 is what would be expected from a general healthy population.
These Measures are Age And Lab Sensitive
Symptom Name | Shannon | Simpson | Chao | Shannon Rank | Simpson Rank | Chao Rank | Obs |
Age: 0-10 | 1.576 | 0.118 | 8788 | 33.1 | 65.2 | 44.8 | 21 |
Age: 20-30 | 1.599 | 0.065 | 11592 | 32.2 | 49.7 | 52.6 | 57 |
Age: 30-40 | 1.579 | 0.06 | 11119 | 31.9 | 43.5 | 52.9 | 153 |
Age: 40-50 | 1.62 | 0.067 | 10433 | 34.8 | 47.1 | 50.5 | 106 |
Age: 50-60 | 1.605 | 0.045 | 11728 | 32.8 | 34.1 | 55.3 | 47 |
Age: 60-70 | 1.488 | 0.033 | 13580 | 28 | 26 | 60.7 | 22 |
SymptomName | Shannon | Simpson | chao | Shannon Rank | Simpson Rank | Chao Rank | observations |
Age: 20-30 | 2.18 | 0.06 | 2360 | 65 | 49.6 | 15.2 | 38 |
Age: 30-40 | 2.116 | 0.06 | 2058 | 61.6 | 50.3 | 13.9 | 69 |
Age: 40-50 | 1.848 | 0.071 | 1724 | 47.8 | 53.9 | 11.7 | 53 |
Age: 50-60 | 1.747 | 0.039 | 2019 | 42.8 | 32.5 | 13.9 | 47 |
Age: 60-70 | 1.926 | 0.058 | 1691 | 52.1 | 43.7 | 11.4 | 75 |
Symptom Name | Shannon | Simpson | chao | Shannon Rank | Simpson Rank | Chao Rank | observations |
Age: 0-10 | 2.293 | 0.134 | 19797 | 64.1 | 67.7 | 69.8 | 52 |
Age: 20-30 | 2.616 | 0.103 | 21549 | 74.8 | 63.2 | 69.1 | 31 |
Age: 30-40 | 2.816 | 0.083 | 16394 | 81.7 | 63.4 | 67.2 | 96 |
Age: 40-50 | 2.89 | 0.09 | 20275 | 84.8 | 62.2 | 74.1 | 53 |
Age: 50-60 | 3.025 | 0.076 | 21956 | 86.6 | 54 | 75.9 | 38 |
Age: 60-70 | 2.596 | 0.077 | 27740 | 72.4 | 51 | 72.6 | 72 |
For anyone wishing to dig further, all of the raw data is on our citizen science site for download. For example, you can compute lab specific percentiles for each symptom.
Symptom Name | Shannon | Simpson | Chao | Shannon Rank | Simpson Rank | Chao Rank | Obs |
Asymptomatic: Minor Health Issues (a few symptoms only) | 1.931 | 0.048 | 4856.983 | 50.7 | 40.5 | 26 | 58 |
Official Diagnosis: Ulcerative colitis | 1.705 | 0.08 | 5547.478 | 35.2 | 54.7 | 27.5 | 23 |
Neurological: Spatial instability and disorientation | 2.049 | 0.072 | 5981.804 | 55.2 | 51.8 | 29.5 | 56 |
Asymptomatic: No Health Issues | 2.012 | 0.066 | 8924.899 | 53.7 | 45.4 | 29.6 | 188 |
Joint: Sudden and severe episodes of pain | 2.185 | 0.058 | 6160.833 | 58.5 | 48.5 | 30.6 | 48 |
Neuroendocrine: Feeling like you have a high temperature | 1.967 | 0.075 | 6704.373 | 50.9 | 53.8 | 32.6 | 75 |
Autonomic: Blurred or tunnel vision after standing | 1.907 | 0.054 | 6777.784 | 49.9 | 42.1 | 33.2 | 51 |
Neuroendocrine Manifestations: abnormal appetite | 2.012 | 0.06 | 7345.455 | 53.2 | 44.8 | 34.1 | 77 |
Neurological: fasciculations | 1.863 | 0.055 | 8333.9 | 47.9 | 43.3 | 34.2 | 70 |
Onset: over 31 years since onset | 1.657 | 0.089 | 7253.219 | 38.7 | 47.2 | 34.6 | 32 |
Neurological: Confusion | 1.918 | 0.067 | 7627.785 | 49.4 | 48.4 | 35 | 93 |
Immune Manifestations: Diarrhea | 1.936 | 0.075 | 12798.57 | 48.9 | 52.4 | 35 | 178 |
Neuroendocrine: Feeling like you have a low temperature | 1.963 | 0.069 | 7193.583 | 51.5 | 48 | 35.3 | 60 |
Neurological-Vision: Blurred Vision | 1.867 | 0.059 | 7616.119 | 46.4 | 44 | 35.4 | 151 |
Autonomic: Graying or blacking out after standing | 2.037 | 0.058 | 7603.086 | 55.9 | 45.3 | 35.5 | 35 |
Neuroendocrine: Chills or shivers | 1.813 | 0.083 | 7818.411 | 45.2 | 52.6 | 35.6 | 56 |
Neuroendocrine Manifestations: recurrent feelings of feverishness | 1.975 | 0.059 | 8146.892 | 51.2 | 48 | 36.2 | 65 |
Neurological: Difficulty processing information (Understanding) | 1.9 | 0.071 | 8249.883 | 48.3 | 48.1 | 36.3 | 197 |
Neuroendocrine Manifestations: Neuralgia | 1.91 | 0.067 | 10588.14 | 47.2 | 49.6 | 36.4 | 44 |
Neurological-Sleep: Chaotic diurnal sleep rhythms (Erratic Sleep) | 2.009 | 0.059 | 11433.45 | 51.6 | 43.4 | 36.5 | 231 |
Neuroendocrine: Temperature fluctuations throughout the day | 1.957 | 0.065 | 7482.103 | 49.8 | 50.3 | 36.5 | 68 |
Neurological: Impairment of concentration | 1.981 | 0.059 | 10024.12 | 51.6 | 43.8 | 36.8 | 345 |
Immune Manifestations: tender lymph nodes | 1.981 | 0.057 | 8632.264 | 51.6 | 43.2 | 36.9 | 110 |
Sleep: Sleeping all day and staying awake all night | 2.07 | 0.074 | 7632.563 | 56.3 | 54.3 | 37.1 | 32 |
Autonomic: Nausea | 1.911 | 0.07 | 8689.976 | 48.8 | 51.7 | 37.1 | 85 |
Neurological: Short-term memory issues | 2.001 | 0.062 | 8048.832 | 52.5 | 45.6 | 37.2 | 220 |
Neurological: Neuropathy | 1.926 | 0.051 | 11019.76 | 48.2 | 41.4 | 37.5 | 71 |
Official Diagnosis: Metabolic Syndrome | 1.748 | 0.067 | 8212.524 | 43.3 | 44.1 | 37.6 | 21 |
DePaul University Fatigue Questionnaire : Fever & Chills | 1.728 | 0.102 | 9503.591 | 40.5 | 56.2 | 37.6 | 22 |
Neuroendocrine: Lack of appetite | 1.946 | 0.064 | 9142.984 | 50.5 | 44.5 | 37.6 | 61 |
Neurological: Executive Decision Making (Difficulty making) | 2.006 | 0.061 | 11185.76 | 52.4 | 44.4 | 37.7 | 289 |
Immune: Sensitivity to smell/food/medication/chemicals | 1.979 | 0.067 | 9401.077 | 50 | 47.4 | 37.8 | 194 |
Immune Manifestations: Genitorinary / Nocturia – Urinary issues | 2.085 | 0.07 | 7840.608 | 56 | 51.7 | 37.8 | 51 |
Neurological: emotional overload | 2.065 | 0.066 | 8548.668 | 54.8 | 49.5 | 37.8 | 196 |
Neuroendocrine Manifestations: Muscle weakness | 1.95 | 0.062 | 8751.741 | 49.6 | 46.2 | 37.9 | 216 |
General: Myalgia (pain) | 2.009 | 0.059 | 13779.4 | 52.4 | 46.3 | 37.9 | 216 |
Onset: 2000-2010 | 1.949 | 0.064 | 8244.574 | 49.8 | 47.3 | 38 | 122 |
Neuroendocrine: Lost or gained weight without trying | 1.993 | 0.07 | 8316.814 | 51.6 | 50.7 | 38.2 | 118 |
Immune Manifestations: new food sensitivities | 1.983 | 0.067 | 9932.414 | 50.6 | 47.7 | 38.2 | 157 |
Onset: Gradual | 2.043 | 0.062 | 13699.92 | 55 | 47.6 | 38.2 | 172 |
Neurological: High degree of Empathy before onset | 1.975 | 0.07 | 8434.465 | 51 | 51.1 | 38.3 | 86 |
Comorbid-Mouth: Dry Mouth | 1.856 | 0.055 | 8600.484 | 45.7 | 41.3 | 38.4 | 124 |
Neuroendocrine Manifestations: subnormal body temperature | 1.999 | 0.057 | 12240.34 | 52.1 | 40.8 | 38.4 | 235 |
Neurological-Vision: Acquired or exertional dyslexia | 1.911 | 0.076 | 8275.381 | 49.7 | 54.1 | 38.5 | 21 |
Neuroendocrine Manifestations: Dry Eye (Sicca or Sjogren Syndrome) | 1.873 | 0.056 | 10422.66 | 45.7 | 42.4 | 38.5 | 136 |
Neurocognitive: Slowness of thought | 1.958 | 0.061 | 12656.61 | 50.6 | 44.9 | 38.6 | 361 |
Neuroendocrine: Sweating hands | 1.91 | 0.091 | 8249.438 | 48.2 | 56.6 | 38.7 | 48 |
Pain: Chest pain | 2.124 | 0.069 | 10019.41 | 58.3 | 52.3 | 38.7 | 54 |
Blood Type: B Positive | 1.753 | 0.085 | 8271.444 | 40.4 | 55.8 | 38.7 | 36 |
Neurological-Vision: inability to focus eye/vision | 1.946 | 0.061 | 12655.22 | 49.6 | 42.1 | 38.8 | 224 |
Neurological: Difficulty reading | 1.966 | 0.066 | 11904.13 | 50.5 | 45.1 | 39 | 283 |
Neurological-Vision: Impaired Depth Perceptions | 1.917 | 0.093 | 9048.091 | 46.3 | 49.7 | 39 | 33 |
Asymptomatic: Live in house with person with probable microbiome dysfunction | 1.954 | 0.057 | 9649.831 | 50.1 | 43.2 | 39 | 71 |
Neurocognitive: Absent-mindedness or forgetfulness | 1.989 | 0.064 | 12091.81 | 51.5 | 45.6 | 39 | 389 |
Pain: Pain or aching in muscles | 1.958 | 0.068 | 12992.8 | 49.7 | 51.1 | 39 | 182 |
Comorbid: Raynaud’s syndrome (Skin discoloration) | 1.917 | 0.052 | 15270.64 | 48 | 44.7 | 39 | 33 |
Autonomic Manifestations: cardiac arrhythmias | 1.955 | 0.054 | 9592.803 | 51.4 | 40.8 | 39 | 61 |
Pain: Myofascial pain | 2.009 | 0.064 | 19489.55 | 53 | 47.8 | 39.1 | 89 |
Neuroendocrine Manifestations: Excessive adrenaline | 2.027 | 0.064 | 8756.423 | 53.6 | 50.5 | 39.1 | 78 |
Official Diagnosis: Inflammatory Bowel Disease (IBD) | 1.906 | 0.093 | 8392.806 | 47.6 | 58.5 | 39.2 | 31 |
Pain: Joint pain | 2.003 | 0.066 | 10602.51 | 51.9 | 50.6 | 39.3 | 178 |
Joint: Stiffness and swelling | 2.041 | 0.059 | 12655.61 | 53.1 | 43 | 39.3 | 246 |
Neurological-Sleep: Vivid Dreams/Nightmares | 2.06 | 0.058 | 9058.67 | 54.6 | 45.4 | 39.3 | 100 |
Infection: Mycoplasma | 2.054 | 0.075 | 11920.39 | 54 | 54 | 39.4 | 111 |
Neurological: Disorientation | 2.102 | 0.077 | 8725.15 | 56.9 | 55 | 39.5 | 80 |
Comorbid-Mouth: Gingivits / Gum Disease | 2.04 | 0.06 | 8021.06 | 55.7 | 47.7 | 39.8 | 67 |
Autonomic Manifestations: Cortisol disorders or irregularity | 2.015 | 0.064 | 8834.639 | 51.8 | 47.6 | 39.8 | 97 |
General: Headaches | 1.991 | 0.06 | 12468.8 | 51.2 | 44.8 | 39.9 | 309 |
Official Diagnosis: Mast Cell Dysfunction | 2.012 | 0.06 | 13066.28 | 51.8 | 43.1 | 39.9 | 208 |
Autonomic: Dizziness or fainting | 1.932 | 0.062 | 8867.455 | 49.6 | 45.5 | 39.9 | 112 |
Immune Manifestations: general malaise | 1.962 | 0.061 | 13005.88 | 50.5 | 44.9 | 40 | 339 |
Comorbid: Hypothyroidism | 1.982 | 0.061 | 12229.72 | 50.9 | 43.5 | 40 | 258 |
Autonomic Manifestations: nausea | 1.862 | 0.067 | 9328.009 | 46.5 | 47.9 | 40.1 | 108 |
Age: 60-70 | 1.997 | 0.057 | 14128.26 | 51.2 | 40.1 | 40.2 | 195 |
Neuroendocrine Manifestations: Rapid muscular fatiguability | 2.024 | 0.063 | 9405.636 | 53.4 | 46.7 | 40.2 | 165 |
Pain: Aching of the eyes or behind the eyes | 1.954 | 0.058 | 11520.93 | 49.6 | 44.7 | 40.3 | 98 |
Immune Manifestations: Alcohol Intolerant | 1.967 | 0.059 | 12012.28 | 49.9 | 41.8 | 40.5 | 303 |
Neurocognitive: Difficulty understanding things | 1.942 | 0.071 | 9540.413 | 49.8 | 49.6 | 40.5 | 189 |
Neurological-Vision: photophobia (Light Sensitivity) | 2.004 | 0.063 | 12593.74 | 51.2 | 44.7 | 40.5 | 276 |
Onset: less than 16 years since onset | 1.954 | 0.057 | 8948.205 | 49.7 | 46.3 | 40.7 | 83 |
Autonomic Manifestations: irritable bowel syndrome | 1.963 | 0.063 | 13226.9 | 50.8 | 44.2 | 40.8 | 378 |
Neurocognitive: Feeling disoriented | 1.976 | 0.065 | 9168.405 | 50.8 | 49.3 | 40.8 | 126 |
Comorbid-Mouth: TMJ / Dysfunction of the temporomandibular joint syndrome | 1.986 | 0.057 | 13336.82 | 50.9 | 40.2 | 40.8 | 223 |
Autonomic Manifestations: palpitations | 1.876 | 0.064 | 9256.654 | 47 | 45.9 | 40.9 | 133 |
Infection: Human Herpesvirus 6 (HHV6) | 2.056 | 0.068 | 14020.15 | 54.2 | 49.5 | 40.9 | 149 |
Neuroendocrine Manifestations: worsening of symptoms with stress. | 1.989 | 0.061 | 10591.72 | 51.1 | 44.9 | 40.9 | 414 |
Autonomic Manifestations: Postural orthostatic tachycardia syndrome (POTS) | 1.927 | 0.067 | 9603.289 | 48.8 | 45.2 | 40.9 | 128 |
Onset: less than 04 years since onset | 2.034 | 0.073 | 8956.183 | 54.1 | 53.2 | 40.9 | 71 |
Immune Manifestations: Abdominal Pain | 1.947 | 0.068 | 14265.76 | 49.4 | 46.5 | 41.1 | 326 |
Neurological: Word-finding problems | 2.057 | 0.064 | 10858.39 | 53.5 | 46.9 | 41.2 | 289 |
Neurological-Audio: hypersensitivity to noise | 1.958 | 0.064 | 11562.53 | 49.7 | 45.7 | 41.2 | 347 |
Immune Manifestations: recurrent flu-like symptoms | 2.001 | 0.066 | 15145.96 | 51.8 | 48.4 | 41.2 | 124 |
Neuroendocrine: Alcohol intolerance | 1.951 | 0.06 | 13204.94 | 49.6 | 41.8 | 41.3 | 278 |
Post-exertional malaise: Rapid muscular fatigability, | 2.024 | 0.06 | 9815.645 | 52.8 | 44.1 | 41.4 | 169 |
Neurocognitive: Problems remembering things | 1.99 | 0.065 | 11390.81 | 51.3 | 46.9 | 41.4 | 415 |
Comorbid: Constipation and Explosions (not diarrohea) | 2.014 | 0.062 | 14823.47 | 52.2 | 41.9 | 41.5 | 170 |
Neuroendocrine Manifestations: intolerance of extremes of heat and cold | 1.987 | 0.064 | 9946.112 | 50.9 | 47.3 | 41.5 | 249 |
Pain: Eye pain | 1.978 | 0.046 | 14356.53 | 51 | 37.8 | 41.5 | 57 |
Neurological-Audio: Tinnitus (ringing in ear) | 1.96 | 0.061 | 13969.85 | 49.5 | 44.8 | 41.5 | 392 |
Blood Type: A Negative | 1.87 | 0.06 | 8845 | 47.9 | 42.9 | 41.5 | 24 |
Neurocognitive: Unable to focus vision and/or attention | 2.01 | 0.065 | 12425.26 | 52 | 45.7 | 41.6 | 302 |
Other: Sensitivity to mold | 2.043 | 0.067 | 9442.872 | 53.5 | 50.5 | 41.7 | 141 |
Neurocognitive: Can only focus on one thing at a time | 1.981 | 0.06 | 11138.71 | 50.7 | 45 | 41.7 | 394 |
Neurological: Cognitive/Sensory Overload | 1.988 | 0.067 | 10058.01 | 50.7 | 49.4 | 41.7 | 219 |
Immune Manifestations: medication sensitivities. | 2.092 | 0.061 | 13364.06 | 55.5 | 44.5 | 41.7 | 142 |
Neurocognitive: Difficulty paying attention for a long period of time | 2.002 | 0.064 | 11330.78 | 52 | 46.8 | 41.8 | 429 |
Immune Manifestations: hives | 1.973 | 0.056 | 10904.28 | 50.2 | 38.6 | 41.9 | 46 |
Onset: Sudden | 1.704 | 0.068 | 8797.214 | 39.9 | 47.7 | 41.9 | 70 |
Autonomic Manifestations: exertional dyspnea | 1.771 | 0.061 | 9788.978 | 45.3 | 39.9 | 42 | 46 |
Comorbid: Mold Sensitivity / Exposure | 2.072 | 0.066 | 9522.613 | 54.2 | 49.7 | 42.1 | 137 |
Sleep: Need to nap daily | 1.955 | 0.061 | 9768.217 | 49.7 | 46.3 | 42.1 | 129 |
Neuroendocrine Manifestations: sweating episodes | 2.074 | 0.071 | 13323.83 | 54.6 | 47.9 | 42.1 | 183 |
Post-exertional malaise: Inappropriate loss of physical and mental stamina, | 2.034 | 0.063 | 9683.478 | 52.7 | 46.7 | 42.1 | 255 |
Autonomic Manifestations: urinary frequency dysfunction | 2.023 | 0.07 | 8986.062 | 53.6 | 49.5 | 42.2 | 113 |
Neurocognitive: Difficulty expressing thoughts | 1.996 | 0.074 | 10247.57 | 51.8 | 49.4 | 42.2 | 232 |
General: Fatigue | 1.971 | 0.058 | 12031.46 | 50.6 | 44.5 | 42.3 | 554 |
Immune: Flu-like symptoms | 2.032 | 0.067 | 17995.01 | 53.2 | 47.3 | 42.3 | 116 |
Neuroendocrine Manifestations: marked weight change | 2.115 | 0.069 | 13678.23 | 55.3 | 50.2 | 42.3 | 119 |
Comorbid-Mouth: Bruxism – Jaw cleanching / Teeth grinding | 2.005 | 0.062 | 13571.29 | 51.6 | 43.2 | 42.3 | 318 |
Neuroendocrine: Feeling hot or cold for no reason | 1.985 | 0.067 | 10345.56 | 50.5 | 48.5 | 42.4 | 158 |
Autonomic: Ocassional Tachycardia (Rapid heart beat) | 1.908 | 0.067 | 9478.579 | 48.1 | 49.2 | 42.4 | 121 |
Gender: Female | 2 | 0.055 | 9736.427 | 51.3 | 43.3 | 42.4 | 372 |
Immune: Recurrent Sore throat | 1.961 | 0.063 | 11439.67 | 49.5 | 45.9 | 42.5 | 134 |
Neurological-Sleep: Sleep Apnea | 2.148 | 0.066 | 19898.1 | 58.2 | 52.3 | 42.6 | 103 |
Comorbid: Snoring (NOT Sleep Apnea | 2.031 | 0.059 | 14436.91 | 52.7 | 41.6 | 42.6 | 185 |
Sleep: Waking up early in the morning (e.g. 3 AM) | 2.057 | 0.062 | 12919.95 | 53.3 | 45 | 42.7 | 349 |
Neuroendocrine Manifestations: marked diurnal fluctuation | 2.115 | 0.06 | 10086.79 | 58 | 45.6 | 42.7 | 24 |
Immune Manifestations: Mucus in the stool | 2.045 | 0.078 | 14352.74 | 52.9 | 51.6 | 42.7 | 176 |
Neuroendocrine Manifestations: cold extremities | 1.961 | 0.061 | 14395.2 | 49.6 | 45.3 | 42.8 | 267 |
Neuroendocrine Manifestations: loss of adaptability | 1.963 | 0.065 | 22154.92 | 51 | 47.4 | 42.9 | 62 |
Neurological-Sleep: Night Sweats | 2.071 | 0.065 | 13304.88 | 53.3 | 46.3 | 42.9 | 141 |
Pain: Sensitivity to pain | 2.049 | 0.072 | 12457.74 | 53.1 | 51.1 | 42.9 | 181 |
Immune Manifestations: Bloating | 1.977 | 0.063 | 13344.09 | 50.3 | 45 | 42.9 | 504 |
Post-exertional malaise: Difficulty reading after mild physical or mental activity | 1.991 | 0.065 | 10766.63 | 51.2 | 47 | 42.9 | 192 |
Onset: less than 08 years since onset | 2.054 | 0.059 | 9535.966 | 54.9 | 43.3 | 43 | 58 |
Neurological-Sleep: Insomnia | 2.03 | 0.061 | 14473.17 | 52.3 | 44.6 | 43 | 339 |
Neuroendocrine: Cold limbs (e.g. arms, legs hands) | 1.969 | 0.067 | 12977.49 | 50.6 | 46.4 | 43 | 191 |
Sleep: Unrefreshed sleep | 1.989 | 0.059 | 12300.45 | 51.3 | 44.1 | 43 | 479 |
Immune Manifestations: Constipation | 2.004 | 0.059 | 14280.13 | 52.1 | 43.2 | 43 | 395 |
General: Depression | 2.11 | 0.067 | 12559.02 | 55.7 | 49.1 | 43 | 278 |
Official Diagnosis: Irritable Bowel Syndrome | 1.999 | 0.065 | 14433.07 | 51.3 | 45 | 43.1 | 349 |
Comorbid: Multiple Chemical Sensitivity | 2.053 | 0.056 | 12104.99 | 53.9 | 41.3 | 43.1 | 131 |
Neuroendocrine Manifestations: Air Hunger | 1.931 | 0.058 | 9873.469 | 48.8 | 44.5 | 43.1 | 81 |
Sleep: Daytime drowsiness | 2.018 | 0.065 | 14393.73 | 52.2 | 46.6 | 43.1 | 349 |
Autonomic: Shortness of breath | 1.976 | 0.067 | 9794.895 | 50.5 | 47.8 | 43.1 | 124 |
Immune Manifestations: Thick blood / Hypercoagulation | 1.99 | 0.061 | 9581.985 | 51.2 | 46.8 | 43.2 | 66 |
Sleep: Problems falling asleep | 2.031 | 0.065 | 10621.44 | 52.4 | 47.8 | 43.2 | 221 |
Infection: Lyme | 2.029 | 0.057 | 11234.89 | 52.6 | 46.8 | 43.2 | 71 |
Neurological-Sleep: Inability for deep (delta) sleep | 2.097 | 0.071 | 14421.58 | 55.9 | 48.9 | 43.2 | 151 |
Onset: less than 02 years since onset | 1.896 | 0.063 | 9318.657 | 46.1 | 46.3 | 43.3 | 67 |
Onset: 2010-2020 | 1.99 | 0.064 | 12458.24 | 50.4 | 44.7 | 43.4 | 325 |
Age: 10-20 | 2.014 | 0.071 | 9357.475 | 53.2 | 49.8 | 43.4 | 40 |
Autonomic Manifestations: light-headedness | 1.988 | 0.07 | 10053.82 | 50.4 | 49.3 | 43.6 | 145 |
Sleep: Problems staying asleep | 2.051 | 0.059 | 13891.49 | 52.9 | 44.1 | 43.7 | 380 |
Autonomic: Irregular heartbeats | 2.047 | 0.068 | 14841.51 | 52.8 | 48 | 43.8 | 141 |
Comorbid: Histamine or Mast Cell issues | 1.969 | 0.059 | 13247.94 | 49.7 | 43.5 | 43.8 | 355 |
Other: Sensitivity to vibrations | 1.929 | 0.044 | 12403.73 | 47.9 | 37.3 | 43.9 | 52 |
Post-exertional malaise: Mentally tired after the slightest effort | 2.018 | 0.063 | 10731.34 | 51.5 | 47.2 | 43.9 | 161 |
Official Diagnosis: Allergic Rhinitis (Hay Fever) | 1.965 | 0.057 | 13655.98 | 49.7 | 41.6 | 43.9 | 232 |
Infection: Epstein-Barr virus | 2.117 | 0.06 | 13402.13 | 55.8 | 45.4 | 44 | 240 |
Infection: Coxsackie | 2.1 | 0.061 | 9782.615 | 57.3 | 47.6 | 44.2 | 39 |
Post-exertional malaise: Rapid cognitive fatigability, | 2.055 | 0.061 | 10488.19 | 53.6 | 46.8 | 44.2 | 212 |
Neurocognitive: Brain Fog | 1.982 | 0.064 | 12153.58 | 50.4 | 46.3 | 44.3 | 597 |
Comorbid: Small intestinal bacterial overgrowth (SIBO) | 2.02 | 0.071 | 13923.31 | 51.2 | 52.7 | 44.4 | 191 |
Immune: Tender / sore lymph nodes | 2.096 | 0.068 | 14842.85 | 53.6 | 46.7 | 44.4 | 111 |
Post-exertional malaise: Worsening of symptoms after mild mental activity | 2.016 | 0.071 | 10902.02 | 51.9 | 51.4 | 44.4 | 165 |
General: Sinus issues with headaches | 2.024 | 0.065 | 13296.41 | 52 | 46.4 | 44.5 | 124 |
Age: 20-30 | 1.974 | 0.07 | 16286.07 | 51.1 | 50.9 | 44.5 | 136 |
Post-exertional malaise: Next-day soreness after everyday activities | 2.104 | 0.064 | 12530.68 | 54.4 | 47.2 | 44.5 | 198 |
Immune Manifestations: Inflammation of skin, eyes or joints | 2.097 | 0.068 | 16251.55 | 54.8 | 51.1 | 44.6 | 228 |
Post-exertional malaise: General | 2.053 | 0.063 | 13379.21 | 53.4 | 48.9 | 44.6 | 243 |
Neurological: Slowed speech | 2.179 | 0.08 | 14630.11 | 57.9 | 49.4 | 44.7 | 108 |
Post-exertional malaise: Post-exertional malaise | 2.072 | 0.06 | 10683.72 | 53.8 | 46.3 | 44.7 | 269 |
Neurological: Joint hypermobility | 2.063 | 0.072 | 15030.99 | 53.3 | 48.8 | 44.8 | 167 |
Neurological: Seasonal Affective Disorder (SAD) | 2.171 | 0.073 | 12976.18 | 58.7 | 49.8 | 44.9 | 127 |
Neuroendocrine Manifestations: Laboured breathing | 2.003 | 0.077 | 11266.07 | 52.1 | 51.8 | 44.9 | 30 |
Autonomic Manifestations: delayed postural hypotension | 2.091 | 0.08 | 10730.32 | 56.3 | 51.3 | 44.9 | 28 |
Infection: Parasite – Other | 2.089 | 0.079 | 13683.44 | 55.4 | 52.2 | 44.9 | 81 |
Autonomic: Heart rate increase after standing | 1.946 | 0.058 | 10621.93 | 50.4 | 44.6 | 44.9 | 145 |
Comorbid: Inflammatory bowel disease | 2.096 | 0.078 | 15575.55 | 54.1 | 51.6 | 45 | 162 |
Immune: Chronic Sinusitis | 1.969 | 0.056 | 9423.875 | 49.4 | 44.9 | 45 | 80 |
Neuroendocrine Manifestations: Paraesthesia (tingling burning of skin) | 2.177 | 0.075 | 15722.67 | 57.3 | 52.5 | 45.1 | 129 |
Immune Manifestations: Hair loss | 2.111 | 0.075 | 17633.89 | 55.2 | 53.5 | 45.1 | 218 |
Onset: 1990-2000 | 2.132 | 0.056 | 30905.83 | 56.2 | 46.3 | 45.1 | 42 |
Post-exertional malaise: Physically drained or sick after mild activity | 2.092 | 0.063 | 11092.9 | 55.3 | 47.1 | 45.1 | 207 |
Neurological-Sleep: Prolonged Sleep | 1.922 | 0.059 | 9645.784 | 48.1 | 41.6 | 45.2 | 37 |
Official Diagnosis: Autism | 2 | 0.081 | 11715.03 | 50.2 | 52.3 | 45.2 | 207 |
Age: 50-60 | 2.013 | 0.049 | 16092.02 | 50.4 | 37 | 45.4 | 142 |
Official Diagnosis: Chronic Fatigue Syndrome (CFS/ME) | 2.046 | 0.052 | 13112.63 | 52.8 | 40.9 | 45.6 | 528 |
Post-exertional malaise: Muscle fatigue after mild physical activity | 2.087 | 0.069 | 12312.77 | 54 | 50.1 | 45.6 | 265 |
Autonomic Manifestations: Orthostatic intolerance | 2.087 | 0.069 | 14092.74 | 54.9 | 49.7 | 45.7 | 164 |
Comorbid: Fibromyalgia | 2.099 | 0.074 | 18779.22 | 55.9 | 53.2 | 46 | 107 |
Neurological: Myoclonic jerks or seizures | 1.982 | 0.053 | 11801.6 | 49.9 | 36.9 | 46 | 50 |
Official Diagnosis: Depression | 2.137 | 0.069 | 13752.19 | 57 | 50.9 | 46 | 99 |
Autonomic Manifestations: bladder dysfunction | 1.947 | 0.064 | 10480.63 | 50.3 | 43.6 | 46.1 | 56 |
Official Diagnosis: Fibromyalgia | 2.042 | 0.071 | 20205.99 | 53.1 | 50.8 | 46.1 | 73 |
Age: 40-50 | 1.989 | 0.073 | 10590.26 | 50.3 | 52.1 | 46.2 | 216 |
Joint: Tenderness | 2.141 | 0.074 | 19366.06 | 56.4 | 51.8 | 46.2 | 173 |
Gender: Male | 2.066 | 0.067 | 14723.23 | 53.5 | 49 | 46.2 | 507 |
Post-exertional malaise: Physically tired after minimum exercise | 2.053 | 0.064 | 13813.96 | 52.7 | 47.5 | 46.2 | 268 |
Post-exertional malaise: Worsening of symptoms after mild physical activity | 2.1 | 0.062 | 12504.35 | 54.6 | 46.6 | 46.4 | 282 |
Immune Manifestations: High Altitude Intolerance | 1.997 | 0.067 | 27233.9 | 51.5 | 48 | 46.5 | 49 |
Official Diagnosis: High Blood Pressure (Hypertension) | 2.149 | 0.079 | 24512.04 | 56.7 | 62.8 | 46.6 | 27 |
Comorbid: High Anxiety | 2.085 | 0.066 | 16472 | 54.3 | 47.4 | 46.6 | 302 |
Blood Type: O Positive | 2.107 | 0.061 | 14366.56 | 54.8 | 43.4 | 46.7 | 262 |
Autonomic: Inability to tolerate an upright position | 2.119 | 0.074 | 16184.94 | 56.3 | 50.2 | 47 | 136 |
Official Diagnosis: Autoimmune Disease | 2.217 | 0.069 | 18238.23 | 59.4 | 49.8 | 47.3 | 184 |
Comorbid: Methylation issues (MTHFR) | 2.158 | 0.066 | 11514.35 | 57.8 | 52 | 47.3 | 113 |
Comorbid: Restless Leg | 2.071 | 0.065 | 10681.73 | 54.5 | 48.8 | 47.4 | 93 |
Official Diagnosis: Mood Disorders | 2.294 | 0.074 | 10430.17 | 62.8 | 57.7 | 47.6 | 54 |
Immune Manifestations: Inflammation (General) | 2.087 | 0.071 | 16527.17 | 54.1 | 51.9 | 47.6 | 277 |
Onset: less than 32 years since onset | 2.053 | 0.063 | 12146.14 | 51.7 | 52.7 | 47.6 | 70 |
Neurological: Dysautonomia | 2.025 | 0.067 | 14155.11 | 51.8 | 46.9 | 47.8 | 156 |
Age: 30-40 | 2.058 | 0.066 | 11989.15 | 53.1 | 49.9 | 48 | 330 |
DePaul University Fatigue Questionnaire : Feeling like you have a temperature | 1.938 | 0.074 | 10889.88 | 47.3 | 55.3 | 48.2 | 43 |
DePaul University Fatigue Questionnaire : New trouble with math | 2.19 | 0.072 | 11232.03 | 54.9 | 57.6 | 48.4 | 35 |
DePaul University Fatigue Questionnaire : Chilled or shivery | 1.931 | 0.071 | 26909.26 | 46.4 | 50.9 | 48.6 | 47 |
DePaul University Fatigue Questionnaire : Sweating hands | 1.859 | 0.091 | 10413.74 | 42.2 | 55.8 | 48.9 | 27 |
Official Diagnosis: COVID19 (Long Hauler) | 1.617 | 0.083 | 10288.01 | 33.5 | 54.3 | 48.9 | 203 |
Comorbid-Mouth: Mouth Sores | 2.146 | 0.066 | 17904.74 | 55 | 45.6 | 48.9 | 70 |
Comorbid-Mouth: Ulcers (mouth) | 1.964 | 0.062 | 11296.41 | 49.6 | 45.9 | 49 | 27 |
Official Diagnosis: Osteoporosis | 1.888 | 0.054 | 10854.14 | 47.1 | 40.8 | 49 | 21 |
Immune: Viral infections with prolonged recovery periods | 2.066 | 0.066 | 11620.85 | 53.6 | 48.8 | 49 | 99 |
Comorbid: Salicylate sensitive | 1.529 | 0.048 | 10532.92 | 30.1 | 37.5 | 49.1 | 36 |
Comorbid: Mood Swings | 1.99 | 0.07 | 11071.23 | 48.6 | 51.5 | 49.4 | 97 |
DePaul University Fatigue Questionnaire : Tingling feeling | 1.705 | 0.072 | 14165.09 | 37.1 | 49.1 | 49.4 | 66 |
Blood Type: O Negative | 1.945 | 0.077 | 11344.33 | 47.7 | 45.4 | 49.5 | 30 |
Comorbid: Electromagnetic Sensitivity (EMF) | 2.224 | 0.065 | 12963.08 | 60.2 | 47.6 | 49.5 | 39 |
Neurological-Sleep: Sleep Reversal | 2.225 | 0.065 | 10837.67 | 60.1 | 53.2 | 49.6 | 33 |
Neuroendocrine Manifestations: Painful menstrual periods | 2.145 | 0.059 | 11232.21 | 57.6 | 45.4 | 49.6 | 57 |
DePaul University Fatigue Questionnaire : Impaired Memory & concentration | 2.037 | 0.069 | 17173.98 | 51.4 | 49.3 | 49.7 | 298 |
Comorbid: Carbohydrate intolerance | 1.961 | 0.061 | 12491.89 | 51.1 | 45.3 | 49.9 | 46 |
DePaul University Fatigue Questionnaire : Frequently get words or numbers in the wrong order | 2.095 | 0.077 | 12878.05 | 53 | 54.6 | 49.9 | 56 |
DePaul University Fatigue Questionnaire : Chest pain | 1.966 | 0.078 | 13689.93 | 47.9 | 55.4 | 50.3 | 27 |
Onset: 1980-1990 | 2.043 | 0.047 | 37107.56 | 53.8 | 36.7 | 50.4 | 32 |
DePaul University Fatigue Questionnaire : Poor Appetite | 1.949 | 0.054 | 14012.92 | 48.5 | 43.7 | 50.5 | 37 |
Official Diagnosis: Attention deficit hyperactivity disorder (ADHD) | 2.081 | 0.078 | 11843.49 | 55.1 | 54.8 | 50.5 | 80 |
Comorbid-Mouth: Periradicular periodontitis inflammatory / chronic lesion around roots of teeth | 2.031 | 0.048 | 11195.24 | 54.4 | 38.9 | 50.6 | 25 |
DePaul University Fatigue Questionnaire : Nausea | 1.856 | 0.074 | 12353 | 44.3 | 51.8 | 50.7 | 63 |
Physical: Eastern European | 1.71 | 0.057 | 11299.21 | 36.8 | 45.9 | 50.8 | 34 |
DePaul University Fatigue Questionnaire : Difficulty reasoning things out | 1.955 | 0.078 | 11585.79 | 48 | 52.9 | 50.9 | 79 |
Joint: Redness | 2.274 | 0.072 | 22181.72 | 61.8 | 50 | 51 | 46 |
DePaul University Fatigue Questionnaire : Blurred Vision | 1.766 | 0.062 | 11329.24 | 39.4 | 45.9 | 51 | 62 |
DePaul University Fatigue Questionnaire : Difficulty falling asleep | 2.024 | 0.08 | 12073.2 | 50.1 | 53.8 | 51 | 133 |
DePaul University Fatigue Questionnaire : Unrefreshing Sleep, that is waking up feeling tired | 2.062 | 0.068 | 16938.43 | 52 | 48.9 | 51.4 | 334 |
DePaul University Fatigue Questionnaire : Racing heart | 1.953 | 0.075 | 22527.55 | 46.7 | 51.5 | 51.4 | 67 |
DePaul University Fatigue Questionnaire : Feel unsteady on feet | 2.026 | 0.057 | 13562.46 | 50.1 | 37.9 | 51.9 | 41 |
DePaul University Fatigue Questionnaire : Fatigue | 2.093 | 0.067 | 16345.97 | 53.2 | 48.8 | 52.1 | 380 |
DePaul University Fatigue Questionnaire : Muscle Pain (i.e., sensations of pain or aching in your muscles. This does not include weakness or pain in other areas such as joints) | 1.968 | 0.072 | 20265.4 | 47.3 | 53.1 | 52.2 | 117 |
General: Heavy feeling in arms and legs | 2.066 | 0.066 | 12763.22 | 53.3 | 48.9 | 52.3 | 104 |
DePaul University Fatigue Questionnaire : Upset stomach | 1.839 | 0.07 | 21705.82 | 42.8 | 47.5 | 52.3 | 79 |
DePaul University Fatigue Questionnaire : Weight change | 2.006 | 0.067 | 11665.45 | 50.4 | 50.4 | 52.5 | 60 |
DePaul University Fatigue Questionnaire : Pain in Multiple Joints without Swelling or Redness | 1.946 | 0.06 | 15796.95 | 46.6 | 49.3 | 52.5 | 74 |
Autism: More Inappropriate Social Interaction | 2.086 | 0.086 | 11929.54 | 54.1 | 54.1 | 52.6 | 24 |
Comorbid: Constipation and Diarrohea (not explosions) | 1.957 | 0.063 | 12512.67 | 48.7 | 48 | 52.7 | 98 |
Comorbid: Sleep Apnea Diagnosis | 2.054 | 0.059 | 41891.19 | 52.7 | 47.8 | 52.7 | 47 |
DePaul University Fatigue Questionnaire : Difficulty retaining information | 2.028 | 0.066 | 18395.93 | 50.8 | 48.6 | 53 | 134 |
DePaul University Fatigue Questionnaire : Mood swings | 2.14 | 0.073 | 12088.44 | 53.3 | 52.2 | 53.2 | 81 |
DePaul University Fatigue Questionnaire : Shortness of breath | 2.031 | 0.072 | 12631.27 | 49.1 | 47.8 | 53.2 | 71 |
DePaul University Fatigue Questionnaire : Difficulty recalling information | 1.98 | 0.067 | 17335.97 | 49 | 49.5 | 53.2 | 152 |
DePaul University Fatigue Questionnaire : Eye pain | 1.881 | 0.051 | 16658.33 | 43.1 | 42.9 | 53.3 | 60 |
DePaul University Fatigue Questionnaire : Headaches | 1.938 | 0.077 | 21075.7 | 46.7 | 51.7 | 53.3 | 97 |
Physical: Long term antibiotics(over 6 months) | 1.953 | 0.082 | 13398.78 | 47.4 | 62.5 | 53.3 | 32 |
Comorbid: Migraine | 2.07 | 0.072 | 18043.71 | 51.2 | 50.5 | 53.4 | 90 |
DePaul University Fatigue Questionnaire : Sore Throat | 1.964 | 0.068 | 14178.04 | 48.4 | 45.3 | 53.5 | 57 |
Physical: Long term (chronic) stress | 1.849 | 0.06 | 19406.05 | 43.2 | 46.1 | 53.5 | 102 |
DePaul University Fatigue Questionnaire : Forgetting what you are trying to say | 2.039 | 0.069 | 18515.81 | 50.3 | 51.4 | 53.6 | 122 |
DePaul University Fatigue Questionnaire : Night sweats | 2.038 | 0.068 | 12137.17 | 49.1 | 47.1 | 53.8 | 46 |
DePaul University Fatigue Questionnaire : Frequently loose train of thought | 2.105 | 0.079 | 12781.15 | 53.7 | 57.3 | 53.8 | 107 |
DePaul University Fatigue Questionnaire : Difficulty following things | 1.995 | 0.08 | 13048.19 | 49 | 53.9 | 54 | 89 |
DePaul University Fatigue Questionnaire : Depression | 2.069 | 0.065 | 19883.27 | 52.7 | 49.7 | 54 | 99 |
DePaul University Fatigue Questionnaire : Allergies | 1.921 | 0.059 | 12296.65 | 47.2 | 45.5 | 54 | 141 |
DePaul University Fatigue Questionnaire : Difficulty comprehending Information | 1.972 | 0.083 | 12479.67 | 47.7 | 54.4 | 54.1 | 121 |
DePaul University Fatigue Questionnaire : Abdomen pain | 1.958 | 0.074 | 22318.12 | 48.2 | 53 | 54.2 | 78 |
DePaul University Fatigue Questionnaire : Hot or Cold spells | 1.935 | 0.065 | 23234.88 | 47.4 | 45.4 | 54.2 | 68 |
Blood Type: A Positive | 2.167 | 0.078 | 16858.1 | 55.6 | 53 | 54.2 | 112 |
DePaul University Fatigue Questionnaire : Muscle weakness | 1.958 | 0.066 | 12329.4 | 47.8 | 49.4 | 54.2 | 123 |
DePaul University Fatigue Questionnaire : Sensitivity to Alcohol | 2.056 | 0.065 | 16243.8 | 51.6 | 46.2 | 54.3 | 98 |
DePaul University Fatigue Questionnaire : Difficulty finding the right word | 2.031 | 0.069 | 17169.14 | 50.8 | 49.8 | 54.4 | 181 |
DePaul University Fatigue Questionnaire : Does physical activity make you feel worse | 2.04 | 0.059 | 17569.78 | 51.4 | 47.2 | 54.5 | 160 |
Physical: Steps Per Day 2000-4000 | 1.898 | 0.057 | 12403.9 | 45.5 | 41.9 | 54.7 | 69 |
Physical: Northern European | 1.847 | 0.062 | 13131.31 | 43.2 | 44.9 | 54.7 | 116 |
DePaul University Fatigue Questionnaire : Temperature lower than normal | 2.05 | 0.06 | 13048.24 | 50.3 | 44.8 | 54.8 | 63 |
Comorbid: Sugars cause sleep or cognitive issues | 1.988 | 0.071 | 14434.56 | 51.9 | 54.8 | 54.8 | 32 |
DePaul University Fatigue Questionnaire : Difficulty staying asleep | 2.135 | 0.065 | 18877.49 | 54 | 48.8 | 54.9 | 141 |
Age: 0-10 | 2.083 | 0.116 | 14335.89 | 55.4 | 62.6 | 54.9 | 87 |
Official Diagnosis: Celiac Disease | 2.036 | 0.08 | 20864.15 | 51.1 | 54.9 | 55 | 34 |
Condition: Acne | 1.926 | 0.055 | 18545.04 | 47.3 | 45.1 | 55.1 | 80 |
DePaul University Fatigue Questionnaire : Walking up early in the morning (e.g. 3AM) | 2.136 | 0.06 | 21152.2 | 54.3 | 47.7 | 55.1 | 102 |
Autism: More deficits in Language Comprehension | 2.118 | 0.093 | 12237.69 | 55.8 | 60.2 | 55.1 | 35 |
DePaul University Fatigue Questionnaire : Confusion/disorientation | 1.905 | 0.075 | 12532.17 | 45.7 | 53.7 | 55.1 | 60 |
DePaul University Fatigue Questionnaire : Need to nap during each day | 1.869 | 0.065 | 25116.62 | 44.8 | 47.6 | 55.3 | 85 |
DePaul University Fatigue Questionnaire : Trouble expressing thoughts | 2.048 | 0.079 | 12709.91 | 50.5 | 56 | 55.3 | 91 |
Autism: More Decrease in Speaking | 1.956 | 0.089 | 11878.78 | 49.8 | 52.4 | 55.4 | 41 |
DePaul University Fatigue Questionnaire : Tense muscles | 1.884 | 0.065 | 21790.03 | 45.6 | 46.9 | 55.5 | 81 |
DePaul University Fatigue Questionnaire : Post-exertional malaise, feeling worse after doing activities that require either physical or mental exertion | 2.044 | 0.064 | 13295.65 | 50.9 | 49.3 | 55.5 | 191 |
Condition: Non-alcoholic Fatty Liver Disease | 1.927 | 0.071 | 12291.76 | 48.4 | 49 | 55.7 | 29 |
DePaul University Fatigue Questionnaire : Rash | 2.141 | 0.07 | 12809.34 | 56 | 49 | 55.9 | 41 |
Condition: Post-Traumatic Stress Disorder | 2.073 | 0.066 | 13172 | 52.1 | 51.4 | 56.1 | 61 |
Autism: More Avoidance of Eye Contact or Poor Eye Contact | 1.954 | 0.087 | 14132.08 | 49.9 | 52.4 | 56.2 | 26 |
Condition: ME/CFS with IBS | 1.941 | 0.064 | 21176.43 | 47.6 | 46.6 | 56.2 | 146 |
DePaul University Fatigue Questionnaire : Absent-mindedness | 2.006 | 0.07 | 19498.31 | 49.6 | 52 | 56.2 | 119 |
Autism: More Behavioral Disturbances | 2.381 | 0.105 | 13815.91 | 66.7 | 63.9 | 56.3 | 23 |
Condition: ME/CFS without IBS | 1.984 | 0.062 | 12510.58 | 49.1 | 45.9 | 56.4 | 89 |
Physical: Paleo Diet | 1.768 | 0.038 | 12820.74 | 40.5 | 31.5 | 56.5 | 23 |
DePaul University Fatigue Questionnaire : Slowness of thought | 1.941 | 0.07 | 12898.63 | 46.8 | 49.4 | 56.6 | 104 |
DePaul University Fatigue Questionnaire : Abnormal sensitivity to light | 2.014 | 0.069 | 14154 | 48.9 | 49.3 | 56.7 | 102 |
Official Diagnosis: Gastroesophageal reflux disease (GERD) | 2.015 | 0.057 | 19077.8 | 51.7 | 44.8 | 56.7 | 65 |
Autism: More Sleep Disturbances | 2.136 | 0.084 | 13719.14 | 56.2 | 55.9 | 56.8 | 36 |
Physical: Good Air Quality | 1.715 | 0.055 | 12224.21 | 37.6 | 42.9 | 56.8 | 29 |
DePaul University Fatigue Questionnaire : Need to have to focus on one thing at a time | 1.949 | 0.061 | 13809.39 | 48.2 | 45.6 | 56.8 | 120 |
Physical: Steps Per Day 4000-8000 | 1.913 | 0.066 | 12897.58 | 45.5 | 47.1 | 57 | 60 |
Autism: More Repetitive Movements | 2.038 | 0.105 | 12658.48 | 53.6 | 62.3 | 57 | 29 |
DePaul University Fatigue Questionnaire : Ringing in the Ears | 1.998 | 0.062 | 20337.6 | 48.4 | 47.5 | 57.2 | 126 |
DePaul University Fatigue Questionnaire : Dizziness | 1.983 | 0.066 | 14162.57 | 46.5 | 48.5 | 57.3 | 65 |
DePaul University Fatigue Questionnaire : Chemical sensitivity | 2.221 | 0.066 | 14833.72 | 57.1 | 48.5 | 57.3 | 79 |
DePaul University Fatigue Questionnaire : Anxiety/tension | 2.037 | 0.067 | 18111.31 | 51.4 | 49.2 | 57.5 | 159 |
Autism: More Intense Focus on One Topic | 2.355 | 0.059 | 15148.77 | 63.7 | 47.7 | 57.5 | 22 |
Physical: Tonsils removed | 2.077 | 0.069 | 13512.92 | 54.6 | 45.4 | 57.7 | 49 |
General: Anhedonia (inability to feel pleasure) | 1.983 | 0.069 | 16245.07 | 47.5 | 54.1 | 57.8 | 27 |
DePaul University Fatigue Questionnaire : Poor hand to eye coodination | 2.329 | 0.07 | 14096.38 | 57.2 | 52.1 | 58 | 21 |
Condition: Psoriasis | 2.174 | 0.08 | 26532.52 | 56.8 | 54.4 | 58.2 | 33 |
Neuroendocrine Manifestations: Poor gut motility | 1.888 | 0.067 | 19063.31 | 44.1 | 48.4 | 58.3 | 133 |
DePaul University Fatigue Questionnaire : Concern with driving | 2.084 | 0.053 | 13892.65 | 53.3 | 43.1 | 58.3 | 40 |
DePaul University Fatigue Questionnaire : Easily irritated | 2.077 | 0.07 | 15797.35 | 52.6 | 51.3 | 58.4 | 142 |
Physical: Work-Sitting | 1.924 | 0.062 | 18699.64 | 46.1 | 45.2 | 58.5 | 139 |
Physical: Breastfed | 2.17 | 0.049 | 16236.93 | 54.1 | 41.1 | 58.8 | 73 |
Neurocognitive: Aphantasia ( inability to visualize mental images) | 1.939 | 0.078 | 14582.46 | 47.7 | 57.4 | 58.8 | 26 |
Official Diagnosis: Hypercholesterolemia (High Cholesterol) | 2.034 | 0.063 | 13217.88 | 50.9 | 48 | 59.2 | 40 |
Condition: Non-Celiac Gluten Sensitivity | 2.019 | 0.057 | 13551.54 | 50.2 | 44.8 | 59.4 | 78 |
DePaul University Fatigue Questionnaire : Slow to react | 2.113 | 0.07 | 14951.93 | 52.7 | 51.2 | 59.4 | 75 |
Condition: Asthma | 2.202 | 0.071 | 14193.31 | 56.9 | 55.2 | 59.5 | 32 |
Physical: Steps Per Day 8000-16000 | 2.18 | 0.063 | 19185.95 | 55.5 | 47.8 | 60.1 | 37 |
Immune Manifestations: Chronic Flatus / Flatulence / gas | 2.058 | 0.071 | 22413 | 52.4 | 49.4 | 60.2 | 98 |
Physical: Amalgam fillings | 2.108 | 0.055 | 29287.59 | 54.2 | 39.9 | 60.4 | 49 |
Condition: Generalized anxiety disorder | 2.183 | 0.077 | 39061 | 57.5 | 54.7 | 60.5 | 45 |
Physical: Appendix removed | 2.159 | 0.052 | 19123.71 | 55.6 | 42 | 60.8 | 28 |
Physical: Pets | 2.122 | 0.068 | 24978.46 | 53.6 | 45.7 | 60.8 | 90 |
DePaul University Fatigue Questionnaire : Does physical activity make you feel better | 1.909 | 0.07 | 20720.16 | 47.4 | 51.9 | 61.3 | 38 |
Autism: High Functioning | 2.383 | 0.061 | 15824.14 | 66.9 | 46.5 | 61.3 | 42 |
Physical: Steps Per Day < 2000 | 1.998 | 0.056 | 16104.52 | 49.7 | 42.5 | 61.5 | 63 |
Comorbid: Panic Attacks | 2.194 | 0.073 | 32169.33 | 57 | 50.3 | 62.3 | 48 |
Official Diagnosis: COVID19 (Fully Recovered) | 2.09 | 0.058 | 15757.67 | 52.4 | 47.1 | 62.4 | 27 |
Condition: Osteoarthritis | 1.892 | 0.057 | 14280.87 | 48.6 | 44 | 63.1 | 23 |
Physical: Organic Diet | 2.131 | 0.071 | 26542.31 | 53.6 | 46 | 63.5 | 65 |
Immune Manifestations: Hyperphagia (abnormally hunger or desire to eat) | 2.01 | 0.051 | 14582.67 | 49.9 | 43.5 | 63.7 | 21 |
Recent Comments