Long COVID [Post COVID Syndrome] is likely an immature variant of Myalgic Encephalomyelitis/Chronic Fatigue Syndrome (ME/CFS). I use the term immature because typically a ME/CFS diagnosis comes 2-10 years after on-set. Between onset and a later static state, the microbiome is a state of constant transition attempting to reach a new stable state.
Doing a search on Pub Med for “Long COVID hypoxia”, we get over 200 hits. Searching for “Long COVID coagulation”, we get over 300 hits! The following are quick notes to sketch out avenues for people treating Long COVID, especially patients with brain fog. I have cited classic ME/CFS literature and matching literature on Long COVID. This is a page in progress and may be updated periodically.
How can Hypoxia happen?
There are many ways, here’s a recap:
- Coagulation Issues: Often it is an inherited coagulation defect that flares as a side-effect of post COVID changes (likely of the microbiome). See Thick Blood, Clots dimension of CFS.
- Coagulation is a complex process and “taking a baby aspirin” is NOT a cure all.
- The defect may be in just one or several of the Roman Numeral items shown below. (From Coagulation Cascade)
- The result is often called “Thick Blood”
- See this Johns Hopkins “What Does COVID Do to Your Blood?“
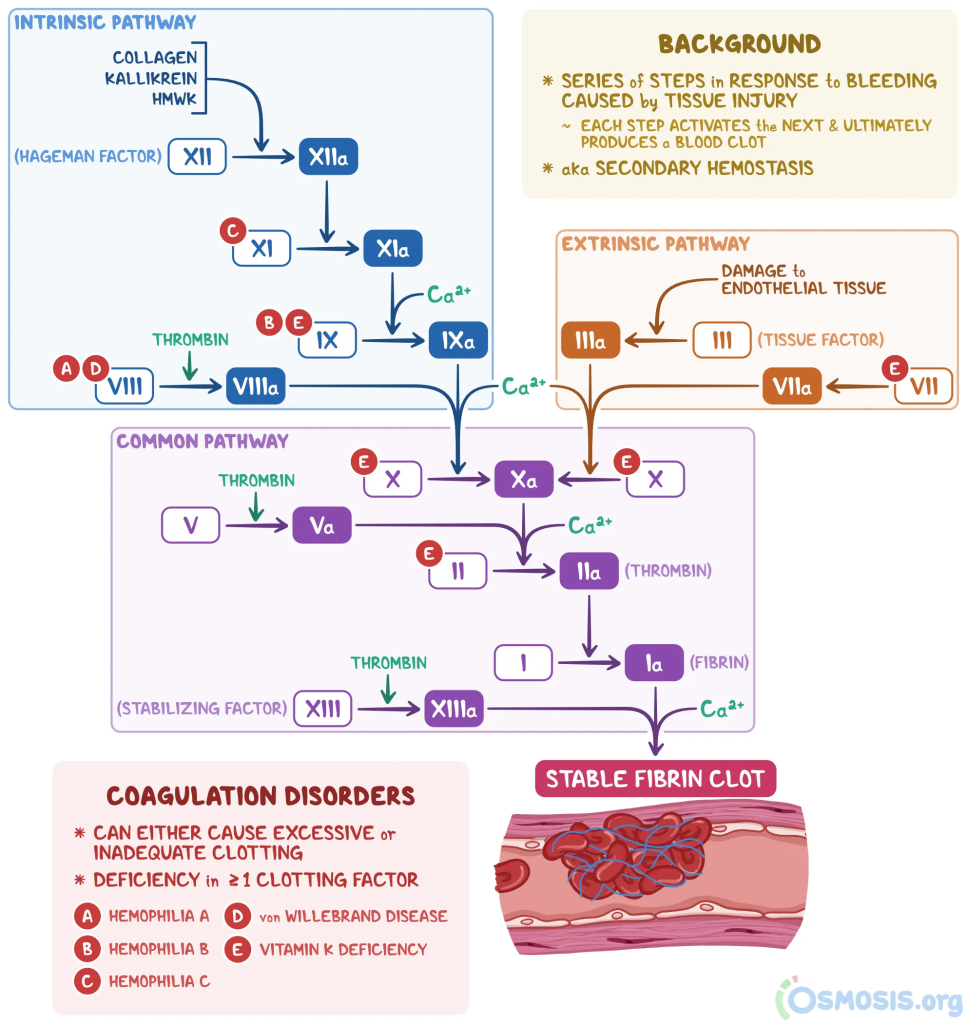
- Issues with blood flow, again many options
- Inflammations of veins (click for more info) and blood vessel damage [NIH]
- Decreased elasticity of red blood cells (click for more info) and similarly misshapen red blood cells
- Decreased blood volume “Circulating Blood Volume in Chronic Fatigue Syndrome [1998]”
- “Studies have reported that COVID-19 patients had significantly more heterogeneous size and deformation of RBCs compared to healthy controls”[SARS-CoV-2 Altered Hemorheological and Hematological Parameters during One-Month Observation Period in Critically Ill COVID-19 Patients]
- Contributing factor: Small heart syndrome [2008] [2009] [2011] [2012] or heart damage [NCBI]
- Hemoglobin issues (Hemoglobin, a form of iron, is what carries oxygen). Some bacteria are iron greedy, reducing the iron available.
- “Iron homeostasis disturbances may persist for more than two months after the onset of COVID-19, which may lead to reduced iron bioavailability, hypoferremia, hyperferritinemia, impaired hemoglobin, and red blood cell synthesis.” [2022]
- “Research has shown that long COVID may be associated with low iron levels and anemia.”
Some Signs of the Above
- Objectively measured abnormalities of blood pressure variability in CFS[2012]
- Lower blood pressure in sleep[2011]
- Lower blood pressure[2009]
- Rapid Heart Beat (Tachycardia) (more info) – because of lower delivery per heart beat, the heart beats more trying to deliver more oxygen
- Small heart on X-Rays
- Low Iron Levels (definition of low should likely be below average, not the lab ranges)
- Saturated O2 level being slightly low
Treatment Options
Hemoglobin improvement
- Lactobacillus acidophilus and Lactobacillus plantarum 299v: When added to iron-fortified milk, it significantly improved red blood cell and hemoglobin levels in anemic children compared to those who consumed iron-fortified milk without the probiotic. [Ameliorating effects of probiotics on alterations in iron homeostasis and inflammation in COVID-19]
- For more details, see this answer.
Coagulation
- Fibrinolytics – Dissolves old Coagulation
- Amyloids, brain fogs and possible treatment approaches.
- Following supplements can inhibit some forms of coagulation
- Omega-3 Fatty Acids
- Selenium
- Magnesium
- Resveratrol
- Rutin
- Ginkgo, ginseng, garlic, ginger, curcumin, salicylates (such as willow bark and aspirin), milk thistle, and jasmine.
Microbiome
- Coagulation Impact:
- The intestinal microbiome potentially affects thrombin generation in human subjects [2020]
- The gut microbiome and thromboembolism [2020]
- The gut microbiota – a modulator of endothelial cell function and a contributing environmental factor to arterial thrombosis. [2019]
- Gut Microbial Metabolite TMAO Enhances Platelet Hyperreactivity and Thrombosis Risk. [2016]
- Identifies: Anaerococcus hydrogenalis, Clostridium asparagiforme, Clostridium hathewayi, Clostridium sporagenes, Escherichia fergusonii, Proteus penneri, Providencia rettgeri, and Edwardsiella tarda
Recent Comments