For a number of years, I taught a 3rd year Artificial Intelligence survey course for Chapman University. Career wise while my “bread and butter” came from software engineering, I have in that profession often done data science, statistics and artificial intelligence for various employers.
Looking at AI systems for the microbiome back in 2015, I went with a model that I had used professionally: Expert System with Fuzzy Logic. An expert system use logic. The term fuzzy logic means that probability is used at decision points.
History Lesson
The first significant use of an expert system in medicine was the development and application of MYCIN in the early 1970s at Stanford University. MYCIN was designed to diagnose bacterial infections, particularly blood diseases, and to recommend appropriate antibiotics, taking into account factors such as patient weight and the specific infection identified. The system was based on a set of about 500 cause-and-effect rules and could explain its reasoning, as well as suggest additional laboratory tests if necessary.
MYCIN demonstrated the potential of artificial intelligence to support clinical decision-making, often matching or even exceeding the diagnostic accuracy of human specialists—achieving around 70% accuracy in controlled tests. Despite its success, MYCIN was never implemented in routine clinical practice, largely due to legal and accountability concerns regarding the use of AI in critical medical decisions.
Other expert systems were developed around the same time, but MYCIN is widely recognized as the first major, influential expert system specifically designed for medical diagnosis and treatment, marking a pivotal milestone in the history of AI in healthcare.
(Doctor) Watson Healthcare Application
IBM Watson’s entry into healthcare began in earnest around 2011, when IBM started developing healthcare-specific applications leveraging Watson’s natural language processing and machine learning capabilities. The system was designed to analyze large volumes of medical data—including electronic health records, medical literature, and clinical guidelines—to assist physicians in making more informed treatment decisions, especially in complex cases like cancer.
Key milestones and applications include:
- 2013: Watson’s first commercial healthcare application was for utilization management decisions in lung cancer treatment at Memorial Sloan-Kettering Cancer Center. This marked the beginning of Watson’s deployment in real-world clinical settings.
- 2016: IBM launched “Watson for Oncology,” a product designed to provide personalized, evidence-based cancer care options to physicians and patients.
Watson was positioned as a tool to bridge knowledge gaps, keep clinicians updated on the latest evidence, and support personalized care by tailoring recommendations to individual patient profiles. However, the initiative faced significant challenges, including high costs, privacy concerns, regulatory hurdles, and mixed adoption by healthcare providers. Notably, the partnership with MD Anderson Cancer Center was discontinued after substantial investment, and Watson for Oncology faced criticism for inconsistent recommendations and limited adaptability to local clinical practices.
Large Language Models
The first significant large language model (LLM) is generally considered to be GPT-1, released by OpenAI in 2018. GPT-1 was relatively small by today’s standards, with only 117 million parameters.
The release of GPT-2 in 2019 (with 1.5 billion parameters), and especially GPT-3 in 2020 (with 175 billion parameters), brought LLMs to much greater prominence and capability. These models, especially GPT-3 and later, are what most consider the foundation for today’s advanced LLM technologies.
Large language models (LLMs) present several well-documented problems and limitations when applied to medicine:
- Hallucinations and Incorrect Information: LLMs can generate plausible-sounding but incorrect or fabricated medical advice, a phenomenon known as “hallucination.” This poses serious risks, especially when users do not verify outputs or lack medical expertise.
- Lack of Medical Domain Optimization: General-purpose LLMs are often not fine-tuned with sufficient medical data, leading to misinterpretations of clinical terminology and context-specific nuances.
- Transparency and Interpretability: The reasoning behind LLM outputs is often opaque (“black box” nature), making it difficult for clinicians and patients to understand or trust the basis for recommendations.
- Algorithmic and Data Bias: Biases in training data or model design can result in unfair or inaccurate recommendations, especially for underrepresented patient groups.
- Automation Bias and Overreliance: Clinicians may become overly reliant on LLM outputs, leading to uncritical acceptance and reduced independent judgment, a phenomenon known as automation bias.
- Limited Regulatory Oversight: There is a lack of clear legal and ethical guidelines governing the use of LLMs in clinical settings, raising concerns about accountability and patient consent.
- Information Completeness and Consistency: LLMs may provide incomplete or inconsistent answers, particularly when faced with complex or rare medical scenarios.
- Privacy and Data Security: The use of sensitive patient data to train or fine-tune LLMs raises concerns about privacy, data security, and compliance with regulations like HIPAA.
- Inequity of Access: Differences in technology access and digital literacy can exacerbate healthcare disparities, limiting the benefits of LLMs for certain populations.
Bottom line: Large Large Models are very questionable for use with the microbiome; while convenient, cheap and heavily hyped – there are so many issues that I view it has having huge legal liability risk dealing with the microbiome in a clinical setting.
Going Forward
A long time Ph.D. friend that attended multiple National Institutes of Standards and Technology conferences with me shared a post with me below. He, like me, have worked in senior positions as Architect and Strategist for firms such as Intel, VMWare, RSA and DELL, while I did time at Microsoft, Amazon, Starbucks and Verizon.
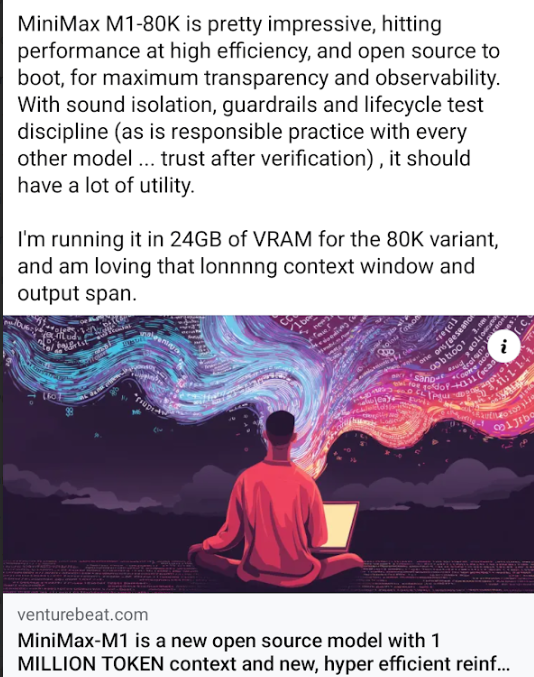
I responded with doing 7 times more data with 1/22 of the memory using my expert system!!!
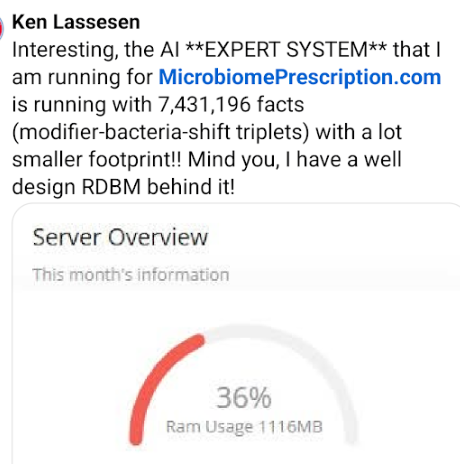
He added:
These LLM-ish technologies cannot approach the efficiency or trustability of an expert system or knowledge based system implementation, and LLMs fall apart under significant ambiguity.LeCun ( world models (e.g. V-Jepa)), Pearl (Causal Models) and a few others are pointing the way to LLM alternatives (not an extension of LLMs).
The next step is at the intersection of Knowledge Representation and Reinforcement Learning , giving us a place to hang prior knowledge, ground truth, dependencies and real logics ( reasoning as opposed to chain of thought).
For those not familiar with these concepts and naively believe that AI is only Large-Language-Models.
Alternative AI Models
Yann LeCun
Yann LeCun is a Turing Award-winning computer scientist, Meta’s Chief AI Scientist, and a professor at New York University. He is best known for pioneering Convolutional Neural Networks (CNNs) in the late 1980s and early 1990s, which revolutionized computer vision and laid the foundation for modern deep learning systems. His work, especially LeNet-5, enabled breakthroughs in handwritten digit recognition and influenced countless AI applications, from facial recognition to autonomous driving.
LeCun is a vocal critic of the current generation of large language models (LLMs), arguing that they lack true reasoning, understanding of the physical world, persistent memory, and planning capabilities. He advocates for the development of world models—AI systems that can observe, interact with, and reason about the world, aiming for human-level intelligence.
V-JEPA (Joint Embedding Predictive Architecture)
JEPA (Joint Embedding Predictive Architecture) is a new paradigm in AI research championed by LeCun. Unlike traditional LLMs, which predict the next token in a sequence, JEPA aims to develop systems that can reason, plan, and interact with the physical world by learning from observation and experience.
JEPA’s core idea is to move beyond mere language processing, focusing instead on building AI architectures that can:
- Understand and model the world (not just text or tokens)
- Reason and plan based on learned representations
- Maintain persistent memory for long-term understanding and context7
This approach is seen as a step toward more robust, general-purpose AI systems that could eventually surpass the limitations of current LLMs.
Judea Pearl (Causal Models)
Judea Pearl is a computer scientist and philosopher renowned for his foundational work on probabilistic and causal reasoning. He introduced the concepts of Bayesian networks and, most notably, the do-calculus for causal inference, which allows researchers to distinguish correlation from causation in complex systems.
Pearl’s causal models provide a mathematical framework for understanding how interventions (e.g., treatments in medicine) affect outcomes, enabling more accurate predictions and explanations in fields like epidemiology, economics, and machine learning. His work has had a profound impact on AI, particularly in areas where understanding cause-and-effect relationships is critical.
Hybrid AI Systems:
- Combining symbolic AI (rule-based reasoning) with neural networks for more robust and interpretable A
Basic Criteria to evaluate an AI Model
Ability to Accurately forecast symptoms and conditions from a sample
A recent educational analysis that I did had a high rate of correctly predicting symptoms. The illustration below are from developing a predictor on a collection of 4000+ samples processed through the same pipeline. The checkmarks are the symptoms that the user confirm having (i.e. a correct prediction).
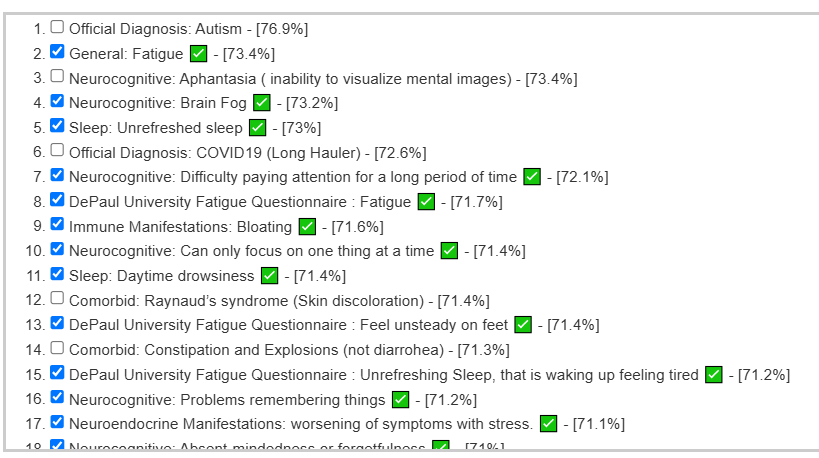
The same person’s earlier sample ( when they had more severe issues) was even more impressive:
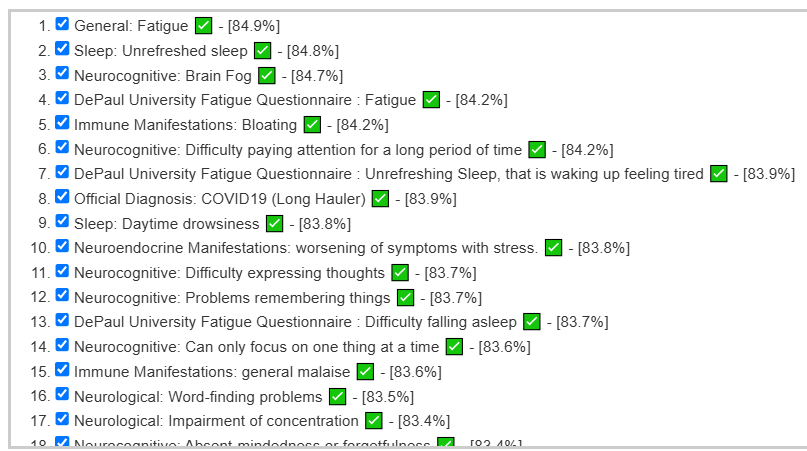
This can also be done using published studies but this has a challenge because of a severe lack of standardization in studies. This translates usually into rare repeatability of results.
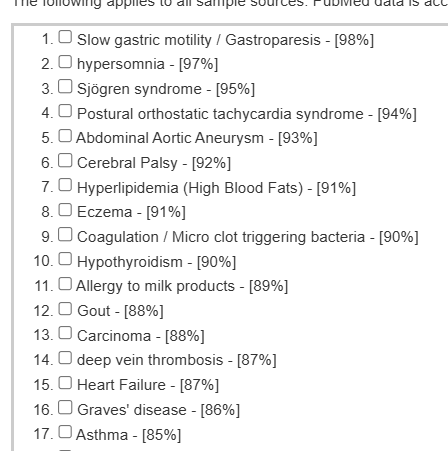
Last week I had a nice session discussing this issue with the Scott whom I cited below.
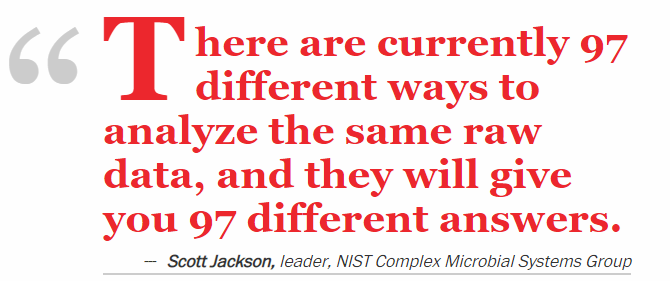
Ability to provide objective evidence of improvement
The ability to forecast gives a natural mechanism of evaluation. If the suggestions from the AI improves the microbiome, then one would expect the values for the forecasts to reduce. An example is shown below (full details). In this example, every single forecast value was decreased from implementing the suggestions from the expert system.
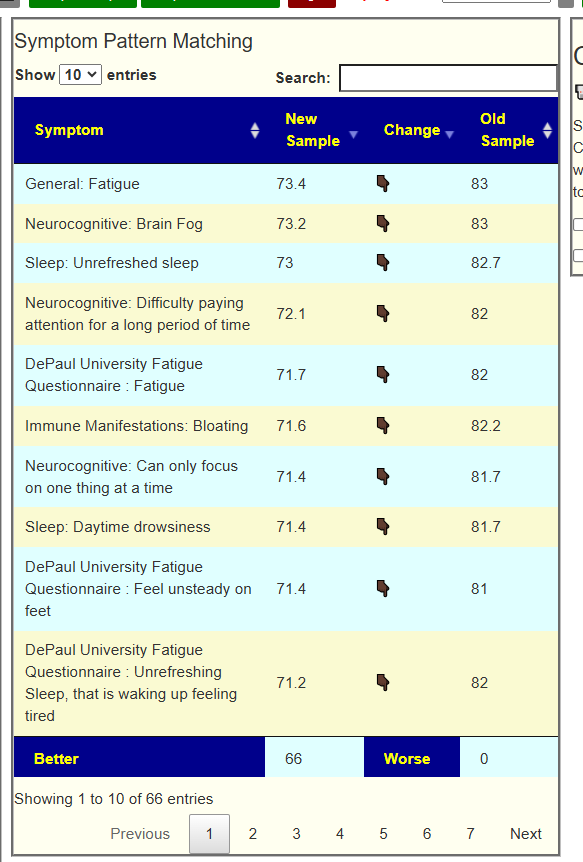
Access to data used above
The microbiome sample data annotated with symptoms that my expert system uses to build algorithms is freely available at my CitizenScience site.
Cheap and sloppy OR Expensive and accurate
Large Language Models (LLMs) are often considered relatively inexpensive to develop compared to earlier AI systems, since they can be trained by scraping vast amounts of text from the internet to identify statistical patterns. In effect, the knowledge these models acquire is based on the aggregate of publicly available information—much of which is uncorroborated or unverified, analogous to hearsay in legal terms.
Legal systems do not accept hearsay as reliable evidence, and this foundational limitation raises significant concerns when LLMs are used in medicine. If an LLM’s output leads to patient harm, its reliance on potentially unreliable or unverified sources could make it difficult for clinicians to defend their actions in malpractice lawsuits, as the standard of care in medicine requires decisions to be based on rigorously validated, evidence-based knowledge.
Expert Systems are typically costly to develop because their construction relies heavily on human expertise to define and encode logic rules. This process involves painstakingly translating medical knowledge and clinical guidelines into a structured set of rules that the system can follow. Furthermore, encoding the necessary facts into the system demands a thorough review of medical literature by subject-matter experts, which is both time-consuming and expensive.
These challenges are compounded by the nature of medical information itself. Critical data—such as detailed clinical findings, research outcomes, or supplementary evidence—are often found in tables, charts, and appendices within medical articles. Traditional expert systems can be engineered to process these structured formats, but this again requires manual effort and expert intervention. In contrast, large language models (LLMs) struggle to reliably extract and interpret information from such tabular data, as their training is primarily based on unstructured text and is less adept at handling complex, structured formats.
As a result, while expert systems offer the advantage of transparent, rule-based reasoning that can be clearly explained and audited, their development remains resource-intensive. This is due to the need for ongoing expert involvement, meticulous data encoding, and specialized handling of non-textual information that LLMs currently manage less effectively.
Bottom Line
My choice (before LLM days) of using Expert Systems appears to still be the most appropriate. While I currently use some Bayesian mathematics in the model (the “Fuzzy”). I will be digging into Judea Pearl’s Causal Models to see what may be effectively incorporated into the existing model.
I have done a video that walks through some of the other issues involved below.
https://www.youtube.com/watch?v=kUnHucfoxL0&t=1s
And some discussion on Expert Systems
https://www.youtube.com/watch?v=yCP33KbFtXM
Recent Comments