Preliminary evaluations have shown strong and accurate forecasting ability. I am working with a Ph.D. in Molecular Genetics and processing his microbiome sample the top forecasts were correct:
- He was a male
- He had no Health Issues
- He was blood type O-positive
- He snored
- He woke up early
This was not from a blood sample, but from a stool sample. The methodology is described in my earlier posts.
The methodology is computing intensive. Unlike many contemporary artificial intelligence engines, it is easy to understand:
- In the entire population(n:10,000), a bacteria like unclassified Clostridiales has 10% of samples reported this bacteria with a mean of 0.3%.
- In the condition / sample population(n:1000) we find that 30% of the samples reported this bacteria with a mean of 4.8%
I used Chi2 to determine significance because significance using means assumes a normal distribution which is a false assumption.
With this methodology it is good to get some indication of what sample sizes for conditions / symptoms is needed (assuming the control is at least the same size). A quick plot informs us that 200 looks like a minimum size with 300+ being desired.
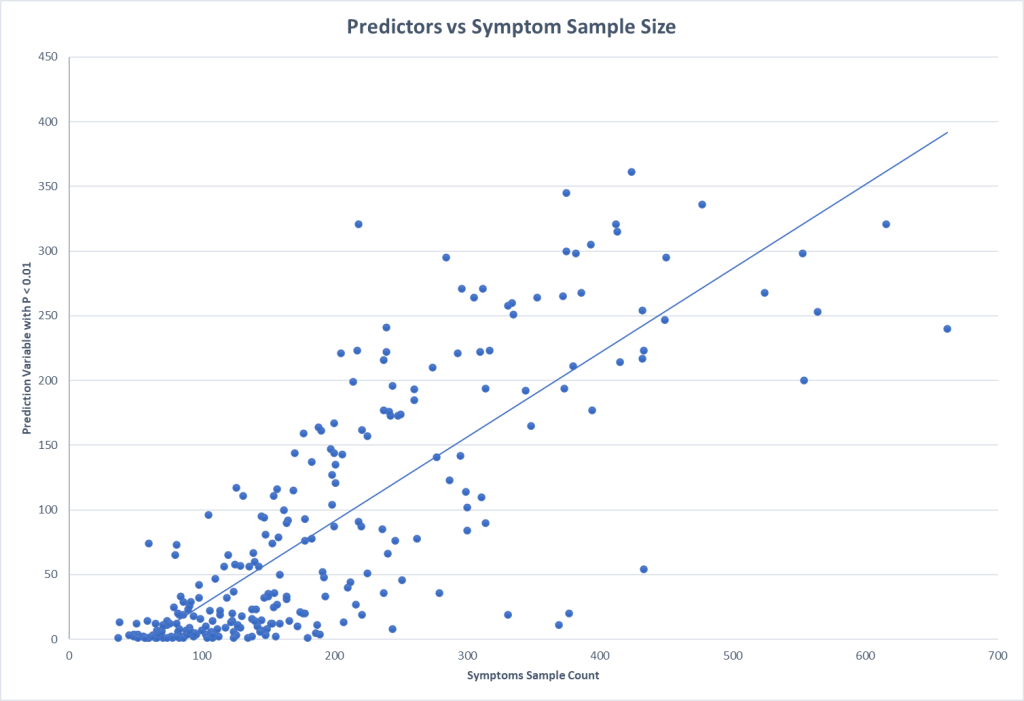
One of the labs that I work with reports percentile ranking on their reports. This makes doing an exploration economical (i.e. cheap). Testing of the symptom/condition group is only needed because the control numbers are gifted you.
This also illustrates that the symptom is not with just one taxa, but the influence of many taxa working in unison to influence the symptom (or be influenced by the condition).
Samples of Symptoms / Conditions
The ones with the most predictors are below.
- Neurocognitive: Absent-mindedness or forgetfulness
- Neurological: Impairment of concentration
- Neurocognitive: Difficulty paying attention for a long period of time
- General: Fatigue
- Autonomic Manifestations: irritable bowel syndrome
- Official Diagnosis: COVID19 (Long Hauler)
- Sleep: Problems staying asleep
- Neurocognitive: Slowness of thought
- Neurological-Sleep: Insomnia
- Sleep: Daytime drowsiness
- Immune Manifestations: Bloating
- Neurocognitive: Problems remembering things
For blood types:
Only two were significant and we have the importance of sample size apparent.
Symptom Name | Sample N | Forecast Variables |
Blood Type: O Positive | 284 | 295 |
Blood Type: A Positive | 126 | 3 |
For Age:
Symptom Name | Sample N | Forecast Variables |
Age: 60-70 | 206 | 143 |
Age: 0-10 | 98 | 42 |
Age: 50-60 | 164 | 33 |
Age: 20-30 | 152 | 12 |
Age: 30-40 | 369 | 11 |
Age: 40-50 | 244 | 8 |
For Gender
Symptom Name | Sample N | Forecast Variable |
Gender: Female | 415 | 214 |
Gender: Male | 554 | 200 |
Bottom Line
From the individual forecast variables, we can compute odds of a match. Consider a simplistic example of three factors
- Factor A uses 5%ile
- Factor B uses 10%ile
- Factor C uses 20%ile
With A,B,C all being true has a 99% chance of being a taxa match (1 – .05 * .1 * .2). This does not mean the person has it, it indicate a significant risk of having it (depending on other factors, DNA, age, gender etc).
Of course, the more forecasting variables, the better the estimate becomes and also the more tolerant the forecast is to variations in the microbiome.
Recent Comments