At present we have many ways to estimate symptoms from a sample. In some cases, we look at what bacteria as a group produces, in other cases just the bacteria. This post is going to look at how well different method behaves. The different approaches are fishing expeditions to see if we can find more predictive analysis tools.
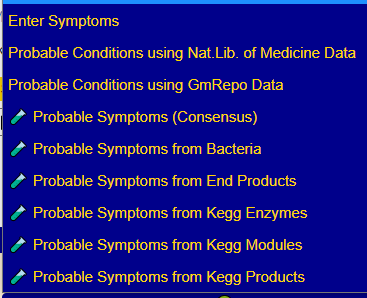
Method
Today, I added the ability to see predicted symptoms against entered symptoms, as shown below
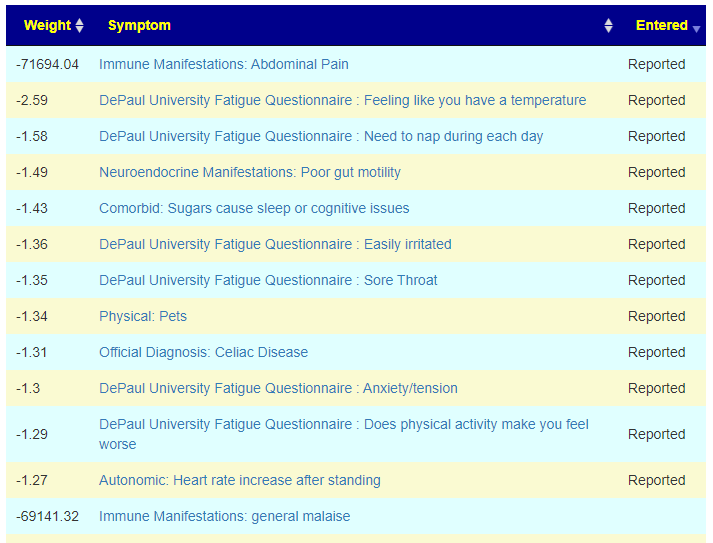
We are going to pick the samples with the most symptoms, one per user and see how well each compares. Sample B had nothing from KEGG — KEGG is works from Species (and this sample lacked any). The nu,mbers below are matches in the top 20 predicted symtp,s/
Method (Top # selected) | A | B | C | D | E | F | G | H | I | J | K | L | M |
Consensus (30) | 11 | 16 | 11 | 6 | 9 | 6 | 3 | 10 | 6 | 7 | 1 | 4 | 4 |
Bacteria (20) | 9 | 16 | 9 | 12 | 11 | 12 | 12 | 2 | 9 | 14 | 4 | 11 | 12 |
End Products (20) | 11 | 8 | 10 | 7 | 4 | 7 | 6 | 5 | 9 | 7 | 3 | 6 | 11 |
KEGG Enzymes (20) | 8 | 0 | 8 | 5 | 8 | 3 | 3 | 8 | 5 | 7 | 1 | 3 | 4 |
KEGG Modules (20) | 13 | 0 | 15 | 10 | 5 | 8 | 9 | 5 | 10 | 7 | 10 | 5 | 9 |
KEGG Products (20) | 7 | 0 | 10 | 7 | 6 | 7 | 10 | 4 | 9 | 9 | 3 | 6 | 7 |
There are challenges with the symptoms entered – namely
- Self-diagnosis
- Many people did not go thru all 485 symptoms, thus many predicted symptoms not matching to reported may be due to incomplete data, or subjective interpretation whether it should be included.
- In the example above, general malaise was not checked but Need to nap during each day and Does physical activity make you feel worse were. The person may not understand malaise or never reviewed this item
What we do find is that every sample had at least one method identifying at least 50% of the person’s symptoms with the highest being 80%, followed by 75%,
Second we find that predicting from bacteria or KEGG Modules had the best performance. In no case was bacteria end products nor KEGG products nor KEGG Enzymes the best. Consensus only once matched the performance of the other two and was often very bad.
Bottom Line
The above shows a strong association of the bacteria (or it’s functions) to various symptoms. It does not prove causality, but causality is my working hypothesis (at least as a catalyst or contributor to symptoms).
This means that modifying bacteria may results in reduction or elimination of many symptoms.
1 thought on “Predicted Symptoms – Performance Review”
Comments are closed.