The nature of data for the microbiome is not a straight line, nor a bell curve. Finding associations is challenging with often poor results I know from years working as a statistician that finding a “magical data transformation” is the key to finding associations. However, a ongoing issue is over-fitting the data when people try formula at random. I have tried a variety of methods from machine learning — with poor results in general.
I put my lateral thinking cap on. Instead of using a defined explicit formula — instead an intrinsic transformation: the percentile of the readings. To do this approach, you need a large sample size – fortunately I have such with over 1500 pairs of data points being common. A similar approach was discussed in Percentile Regression: A Parametric Approach 1978, Journal of the American Statistical Association, but never gained popularity.
This post gives a walk thru of the process being done on 14,374,869 possible associations that we have (excluding symptoms and conditions)
Example
I picked one of my initial good results and will walk thru charts showing how charts change according to the approach. First the raw numbers plotted
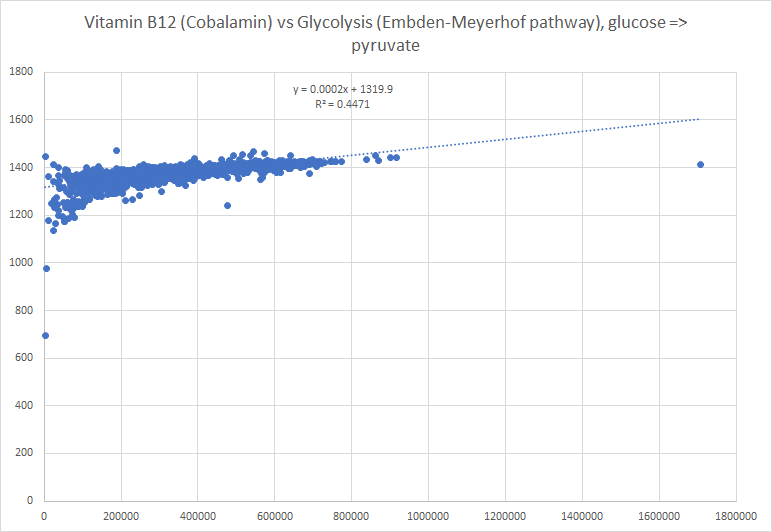
Then we chart of log of the raw numbers (log of the values worked well to determine the Kaltoft-Moldrup normal ranges – KM is based on different moments of the resulting curves)
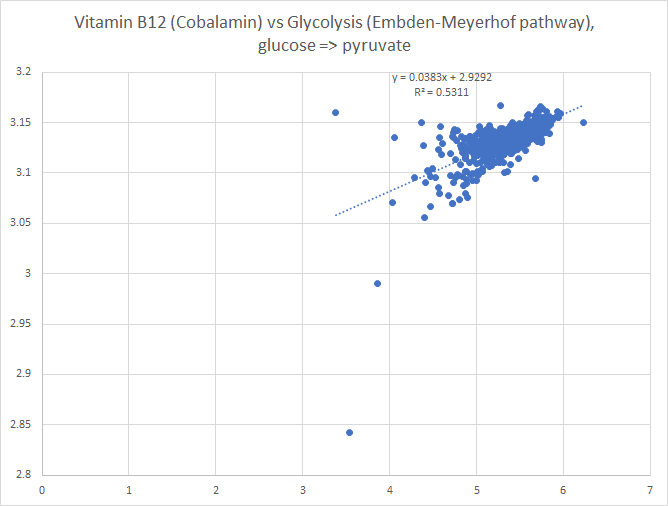
The new way is shown below, using the intrinsic transformation to percentile
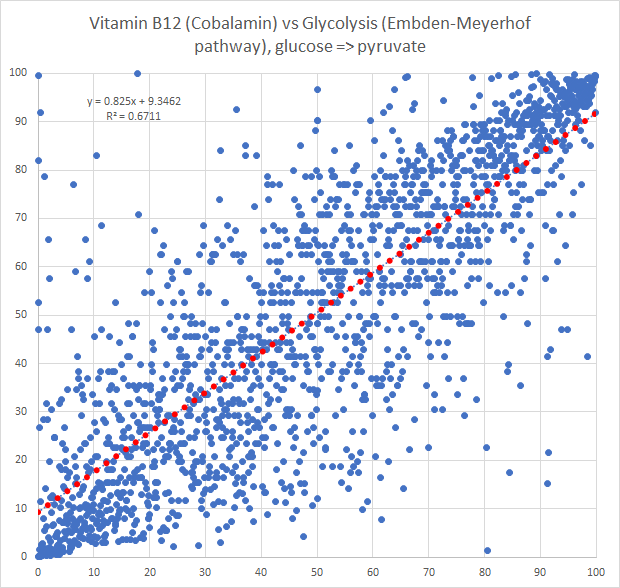
Bottom Line
Finding associations as illustrated above, means we can tease information from our data. For example, for B12 levels, we have a strong association to Glycolysis (Embden-Meyerhof pathway), glucose => pyruvate. This means that the bacteria associated with that is likely associated with B12 production. For example, a few of some 2000+ strains associated with this module.
- Faecalibacterium prausnitzii
- Bacteroides vulgatus
- Bacteroides uniformis
- Parabacteroides distasonis
- Bacteroides caccae
- Bacteroides dorei
- Bacteroides thetaiotaomicron
- Bacteroides ovatus
- Roseburia intestinalis
- Flavonifractor plautii
- Bacteroides fragilis
- Odoribacter splanchnicus
- Alistipes finegoldii
- Eggerthella lenta
Additionally, it means that where there is a relationship between bacteria but we know nothing about how to modify one of the bacteria and something about the other; then we can propose suggestions by association. This will be coming soon to Microbiome Prescription – the citizen science site.
Recent Comments