This month (Feb 2021) there as a major article Structural and Functional Dysbiosis of Fecal Microbiota in Chinese Patients With Alzheimer’s Disease released. I have updated my list of bacteria (with links to source studies),
This post is for caregivers that are interest in low risk treatment that theoretically have a high probability of success and low cost.
Short Summary of Approach
The microbiome produces some 4000+ different chemicals. For many conditions, especially “untreatable”, it appears that imbalances in these chemical mixtures result in cells, including brain cells, malfunctioning.
Some drugs help — and often those drugs were seen to alter the microbiome, correcting some of these shifts. The stupid question is this, if we know the bacteria that are involved — then why not starve or feed to put it into better balance.
IMHO It works! In my 50’s I had a sudden onset of cognitive issues, including memory. A SPECT scan was read as Early Onset Alzheimer’s. I also had another diagnosis. That other diagnosis has a bacteria shift pattern reported in 1998 in Australia. Making changes to alter that pattern caused the cognitive issues to fade and disappear.
Steps
You need to have a microbiome sample (done by taking a little bit of a stool and sending it to a lab). Then the data need to be upload to the free citizen science site, Microbiome Prescription. Not all labs are supported (i.e. they do not make their data available in a suitable format); those that are supported are listed here (with discount codes).
Once the data is uploaded, there are two Quick Suggestions links that generates suggestions using Fuzzy Logic Artificial Intelligence techniques.
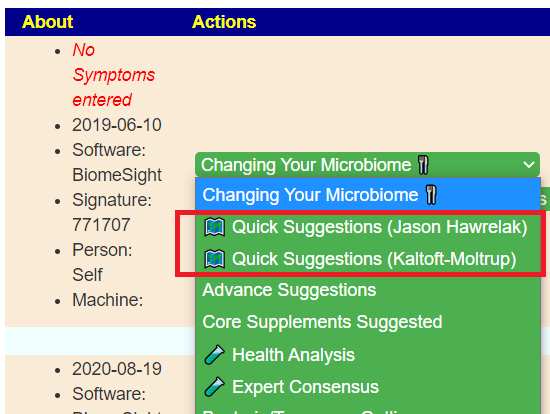
There is a demo logic that show all of the features…. BiomeSight Example Login
There are a lot of tools there, depending on you skill sets and devotion to seeking improvement.
There is a YouTube Channel showing how to use this site and discussion of issues.
Recent Comments