Back Story
Latest microbiome results are in. Seems like my microbiome is stubborn and stuck these last few tests. Wondering if I should just use this test suggestions exclusively or combine with my prior Thorne test
We have two sample – one via BiomeSight and one via Thorne. This post is going to do two things:
- Look at Suggestions – by combining both sets of suggestions using the Uber Consensus
- Look at the differences between the reports.
We also review “which is better”. My focus is clinical application to individuals — not research papers; answer at bottom.
Uber Consensus
The process has become very simple — “Just give me Suggestions!” on both samples and then going to uber consensus as illustrated by the video below.
The result was excellent agreement on suggestion between each set of results. The CSV files are attached below.
Differences between Reports
I compared two things between the reports:
- Percentage of the bacteria in the microbiome
- Percentile of the bacteria in the microbiome
At the Phylum Level
Items less than 100 should be ignored (accuracy of measurement limits). There are a few dramatic differences.
Bacteria Name | Thorne Count | BiomeSight Count |
Firmicutes | 396799 | 529540 |
Actinobacteria | 60610 | 2100 |
Bacteroidetes | 461289 | 448230 |
Proteobacteria | 6095 | 18150 |
Chlorobi | 36 | 429 |
Acidobacteria | 35 | 100 |
Cyanobacteria | 83 | 20 |
Spirochaetes | 85 | 30 |
Verrucomicrobia | 59 | 10 |
Chloroflexi | 77 | 50 |
Tenericutes | 54 | 30 |
Deinococcus-Thermus | 48 | 30 |
Fibrobacteres | 4 | 10 |
Synergistetes | 17 | 20 |
Looking at Percentiles next
Bacteria Name | Thorne %ile | BiomeSight %ile |
Chlorobi | 25 | 84 |
Actinobacteria | 85 | 33 |
Acidobacteria | 34 | 81 |
Spirochaetes | 81 | 36 |
Cyanobacteria | 31 | 1 |
Deinococcus-Thermus | 55 | 29 |
Firmicutes | 14 | 37 |
Chloroflexi | 67 | 50 |
Verrucomicrobia | 14 | 1 |
Tenericutes | 13 | 2 |
Proteobacteria | 10 | 18 |
Synergistetes | 6 | 4 |
Bacteroidetes | 55 | 56 |
Fibrobacteres | 1 | 0 |
We have Bacteroidetes in agreement with both — but for the rest…
At the genus level
Bacteria Name | Thorne Count | BiomeSight Count |
Bacteroides | 180054 | 397640 |
Blautia | 16470 | 107220 |
Roseburia | 16793 | 73640 |
Faecalibacterium | 109196 | 152890 |
Corynebacterium | 43413 | 820 |
Ruminococcus | 9177 | 44170 |
Phocaeicola | 223209 | 199669 |
Parabacteroides | 11855 | 31940 |
Phascolarctobacterium | 6101 | 23980 |
Dorea | 36 | 13000 |
Sutterella | 16 | 11339 |
Oscillospira | 0 | 8250 |
Coprococcus | 6120 | 12589 |
Eggerthella | 6491 | 760 |
Pseudobutyrivibrio | 149 | 5790 |
Lachnospira | 11593 | 6230 |
Prevotella | 954 | 4260 |
Anaerostipes | 9303 | 6310 |
Clostridium | 2039 | 4960 |
Pedobacter | 46 | 2410 |
Odoribacter | 4077 | 2060 |
Bifidobacterium | 2783 | 1019 |
Escherichia | 75 | 1610 |
Porphyromonas | 1372 | 150 |
Mediterraneibacter | 14831 | 13629 |
Bilophila | 6 | 1110 |
Veillonella | 75 | 1160 |
Desulfovibrio | 1900 | 1250 |
Streptococcus | 1477 | 840 |
Acetivibrio | 33 | 470 |
Chlorobaculum | 6 | 429 |
Finegoldia | 1339 | 920 |
Gemella | 17 | 400 |
Enterococcus | 585 | 220 |
Paenibacillus | 376 | 20 |
Mogibacterium | 39 | 370 |
Acetobacterium | 15 | 340 |
Serratia | 47 | 350 |
Eubacterium | 517 | 240 |
Megasphaera | 35 | 290 |
Selenomonas | 52 | 290 |
Bacillus | 248 | 10 |
Caldicellulosiruptor | 11 | 240 |
Campylobacter | 235 | 10 |
Slackia | 16 | 240 |
Sphingobacterium | 48 | 270 |
Caloramator | 10 | 190 |
Staphylococcus | 181 | 10 |
Hathewaya | 8 | 170 |
Peptoniphilus | 656 | 800 |
Peptostreptococcus | 6 | 150 |
Microbacterium | 125 | 10 |
Adlercreutzia | 525 | 620 |
Rhodothermus | 6 | 90 |
Erysipelothrix | 12 | 90 |
Acidaminococcus | 12 | 90 |
Hymenobacter | 80 | 10 |
Negativicoccus | 115 | 50 |
Collinsella | 74 | 10 |
Rhodococcus | 67 | 10 |
Dialister | 25 | 80 |
Anaerococcus | 336 | 390 |
Pseudoclostridium | 8 | 60 |
Moorella | 9 | 60 |
Vibrio | 60 | 10 |
Caldilinea | 1 | 50 |
Brochothrix | 2 | 50 |
Mycobacterium | 67 | 20 |
Neisseria | 57 | 10 |
Pectinatus | 7 | 50 |
Thermoclostridium | 16 | 50 |
Alkaliphilus | 9 | 40 |
Shewanella | 31 | 60 |
Lactobacillus | 57 | 30 |
Leptospira | 4 | 30 |
Deinococcus | 35 | 10 |
Tetragenococcus | 5 | 30 |
Ethanoligenens | 34 | 10 |
Weissella | 10 | 30 |
Gulosibacter | 1 | 20 |
Pseudoclavibacter | 2 | 20 |
Kocuria | 28 | 10 |
Meiothermus | 2 | 20 |
Stenotrophomonas | 28 | 10 |
Symbiobacterium | 3 | 20 |
Devosia | 4 | 20 |
Dysgonomonas | 34 | 20 |
Azoarcus | 21 | 10 |
Leuconostoc | 9 | 20 |
Glaciecola | 1 | 10 |
Turicibacter | 21 | 30 |
Pelotomaculum | 1 | 10 |
Parascardovia | 2 | 10 |
Lentibacillus | 2 | 10 |
Actinopolyspora | 2 | 10 |
Kitasatospora | 2 | 10 |
MLOs | 3 | 10 |
Ochrobactrum | 3 | 10 |
Rickettsia | 3 | 10 |
Luteibacter | 3 | 10 |
Fibrobacter | 4 | 10 |
Pediococcus | 14 | 20 |
Halanaerobium | 6 | 10 |
Dyadobacter | 14 | 10 |
Mycoplasma | 17 | 20 |
Thauera | 9 | 10 |
Lysobacter | 11 | 10 |
Looking at the percentile rankings — the absolute numbers may vary greatly, but what about relative percentiles?
Bacteria Name | Thorne %ile | Biomesight %ile |
Ochrobactrum | 2 | 2 |
Actinopolyspora | 1 | 1 |
Halanaerobium | 1 | 1 |
MLOs | 1 | 1 |
Glaciecola | 1 | 1 |
Lentibacillus | 1 | 1 |
Pelotomaculum | 1 | 1 |
Parascardovia | 1 | 1 |
Luteibacter | 1 | 1 |
Phocaeicola | 89 | 89 |
Rickettsia | 1 | 0 |
Pediococcus | 10 | 9 |
Fibrobacter | 2 | 0 |
Mycoplasma | 5 | 3 |
Alkaliphilus | 1 | 3 |
Finegoldia | 85 | 88 |
Kitasatospora | 3 | 0 |
Thauera | 5 | 1 |
Streptococcus | 55 | 50 |
Turicibacter | 12 | 17 |
Peptoniphilus | 64 | 58 |
Hathewaya | 1 | 8 |
Clostridium | 18 | 11 |
Desulfovibrio | 61 | 69 |
Eubacterium | 38 | 46 |
Symbiobacterium | 1 | 9 |
Enterococcus | 88 | 79 |
Sphingobacterium | 13 | 23 |
Pseudoclavibacter | 1 | 11 |
Anaerococcus | 72 | 83 |
Eggerthella | 98 | 86 |
Gulosibacter | 0 | 12 |
Lactobacillus | 23 | 11 |
Bifidobacterium | 55 | 43 |
Leuconostoc | 2 | 14 |
Shewanella | 35 | 47 |
Prevotella | 50 | 63 |
Corynebacterium | 99 | 86 |
Collinsella | 13 | 0 |
Oscillospira | 0 | 16 |
Faecalibacterium | 49 | 65 |
Meiothermus | 1 | 17 |
Caloramator | 1 | 19 |
Coprococcus | 39 | 57 |
Lysobacter | 18 | 0 |
Odoribacter | 81 | 63 |
Adlercreutzia | 63 | 81 |
Pedobacter | 13 | 31 |
Dyadobacter | 20 | 1 |
Dysgonomonas | 24 | 4 |
Mediterraneibacter | 69 | 90 |
Devosia | 1 | 22 |
Acetivibrio | 5 | 27 |
Thermoclostridium | 9 | 32 |
Ethanoligenens | 25 | 1 |
Dialister | 11 | 35 |
Veillonella | 16 | 41 |
Pectinatus | 1 | 27 |
Porphyromonas | 88 | 62 |
Moorella | 1 | 28 |
Negativicoccus | 66 | 39 |
Lachnospira | 51 | 21 |
Rhodothermus | 1 | 32 |
Tetragenococcus | 1 | 32 |
Acetobacterium | 3 | 34 |
Anaerostipes | 65 | 96 |
Bilophila | 1 | 33 |
Ruminococcus | 14 | 47 |
Weissella | 2 | 35 |
Parabacteroides | 42 | 75 |
Acidaminococcus | 4 | 39 |
Pseudoclostridium | 1 | 37 |
Leptospira | 1 | 42 |
Serratia | 34 | 75 |
Slackia | 4 | 45 |
Phascolarctobacterium | 56 | 97 |
Erysipelothrix | 4 | 46 |
Sutterella | 1 | 46 |
Bacteroides | 39 | 87 |
Roseburia | 43 | 91 |
Escherichia | 28 | 77 |
Selenomonas | 21 | 73 |
Deinococcus | 54 | 1 |
Megasphaera | 18 | 72 |
Brochothrix | 1 | 56 |
Kocuria | 58 | 2 |
Mogibacterium | 17 | 74 |
Stenotrophomonas | 63 | 3 |
Azoarcus | 61 | 0 |
Caldilinea | 0 | 61 |
Caldicellulosiruptor | 2 | 64 |
Mycobacterium | 87 | 24 |
Hymenobacter | 68 | 1 |
Blautia | 5 | 73 |
Paenibacillus | 87 | 19 |
Neisseria | 69 | 0 |
Pseudobutyrivibrio | 25 | 95 |
Campylobacter | 75 | 1 |
Gemella | 4 | 82 |
Peptostreptococcus | 1 | 81 |
Chlorobaculum | 1 | 84 |
Staphylococcus | 85 | 0 |
Vibrio | 91 | 2 |
Bacillus | 92 | 1 |
Rhodococcus | 91 | 0 |
Dorea | 1 | 93 |
Microbacterium | 94 | 1 |
Bottom Line
I have never had much belief in the absolute accuracy of the bacteria named or the count. Why? Simple, I understand the statistical process being used and its weakness. I will leave arguments over “which is better” and “which is accurate” to others.
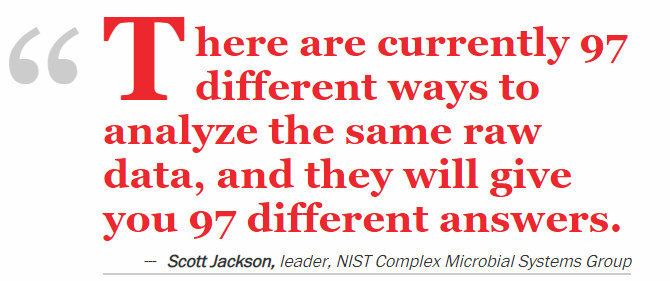
My focus and concern is to improve the microbiome. With sparse data and the great complexity involved, I am actually very pleased that the suggestions are in agreement. The suggestions are computed using fuzzy logic expert systems. The noise in the data and the statistical processes involved seem to be smoothed out by this Artificial Intelligence engine approach.
Score: Labs: -2, Microbiome Prescription 2
Which is better?. My focus is clinical application to individuals, both give similar suggestions using the Fuzzy Logic Expert System. There is no difference in that sense.
Recent Comments