Foreword
This post started out with a title of “Post-Acute COVID-19 Syndrome vs Myalgic Encephalomyelitis – Similarities and Differences“. It scope was pretty obvious — compare microbiome shifts from these two sibling conditions. Expectations was a bland informational review.
The result was calling into question the typical assumption that we could isolate symptoms and conditions to specific bacteria. I follow the statistics and discovered that you can get a magnitude better statistical significance by moving beyond bacteria. In coming weeks, I hope to code up suggestions AI based on this enlightenment.
My starting point
In my last post, Long COVID – an update, I did a comparison between the citizen science data and the literature published on the US Library of Medicine. In terms of symptoms, these two appear the same — but at the microbiome (and enzyme levels) how similar are they?
- Post-Acute COVID-19 Syndrome (PCAS), also known as Long COVID
- Myalgic Encephalomyelitis (ME), also known as Chronic Fatigue Syndrome (CFS)
One frustrating aspect of many studies on the US Library of Medicine for many conditions is simple: results are not replicated in subsequent studies for the same condition. Historically I have viewed this as a result of different equipment and different reference libraries. In many cases the bacteria deemed significant are often different and when they did report the same bacteria, they report opposite shifts!
This post explores some of these issues, and came to an interesting conclusion.
Study Caveats
The studies on the US Library of Medicine compare people with the condition to healthy controls. With the citizen science data that is almost impossible to do. If a person has gotten a microbiome test, they likely have some condition(s) and thus are not healthy controls!!
This is not all bad. It means that when we find things that are statistically significant they are differentiators against other people with microbiome issues. That is, how are people with ME different than people with FM and IBS. Conceptually, we are more likely to identify the key features for these conditions and not key features for auto-immune conditions or a gut disturbance in general. It is a nuisance difference, but may be a very important nuisance.
Comparison that we will review are from:
- US Pubmed — bacteria reported by both with direction
- KEGG Enzymes shifts from Citizen Science (using only Biomesight data)
- Bacteria shifts from Citizen Science (using only Biomesight data)
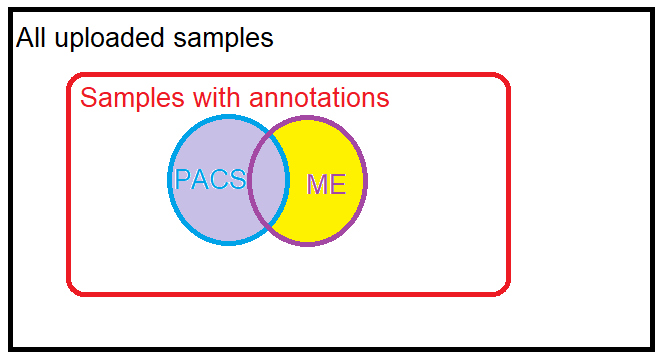
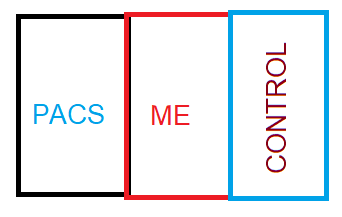
For citizen science we may have many uploaded samples annotated both with PCAS and ME. To resolve this conflict, ME will contain only samples with ME and without PCAS. Both ME and PCAS have many, many comorbid symptoms which may also come into play. Many of the pure ME samples are before COVID swept the world, hence relatively clean. PCAS are more recent samples.
For PACS citizen science data, we have only significance difference identified from Biomesight data, hence we will compare those only.
Scope | ME | PACS | Same |
US National Library of Medicine | 68 | 233 | 25 |
Enzymes – Citizen Science with p < 0.001 | 228 | 199 | 31 |
Bacteria – Citizen Science | 109 | 36 | 0 |
I must admit that finding no bacteria in common with the same lab and the same reference library was a little bit of a surprise. One explanation is that microbiome dysfunctions evolve over time. People with PACS have had it less then 3 years, likely an average of just 1 year. People with ME has had it often for 30+ years. Comparing the two may be similar to comparing a one bottle of grape juice to a bottle of vintage wine.
Details for Common Bacteria from US National Library of Medicine
In the table below: H indicates High, L indicates Low.
Note that Bacteroides are reported high and low in different studies, suggesting there are subsets of each condition
tax_rank | tax_Name | Direction |
class | Bacteroidia | H |
family | Bacteroidaceae | H |
family | Clostridiaceae | H |
family | Lachnospiraceae | L |
genus | Anaerostipes | L |
genus | Bacteroides | H |
genus | Bacteroides | L |
genus | Bifidobacterium | L |
genus | Coprobacillus | H |
genus | Coprococcus | L |
genus | Dorea | L |
genus | Eggerthella | H |
genus | Enterococcus | H |
genus | Faecalibacterium | L |
genus | Lactobacillus | L |
genus | Streptococcus | H |
genus | Turicibacter | H |
order | Eubacteriales | L |
phylum | Bacteroidetes | H |
phylum | Firmicutes | L |
phylum | Fusobacteria | H |
species | Anaerobutyricum hallii | L |
species | Enterocloster bolteae | H |
species | Faecalibacterium prausnitzii | L |
species | Ruminococcus gnavus | H |
Details for Shared Enzymes with p < 0.001
In recent posts for conditions comorbid with ME, PACS, I found that enzyme analysis had greater statistical significance than bacteria. All of these posts reported higher enzyme levels were significant with these conditions.
The result for items shared that had p < 0.001 was almost overwhelming!
ECKey | EnzymeName |
1.1.1.292 | 1,5-anhydro-D-mannitol:NADP+ oxidoreductase |
1.12.98.4 | H2:polysulfide oxidoreductase |
1.7.2.2 | ammonia:ferricytochrome-c oxidoreductase |
1.8.7.3 | CoB,CoM:ferredoxin oxidoreductase |
1.8.98.4 | CoB,CoM,ferredoxin:coenzyme F420 oxidoreductase |
1.8.98.5 | CoB,CoM,ferredoxin:H2 oxidoreductase |
1.8.98.6 | coenzyme B,coenzyme M,ferredoxin:formate oxidoreductase |
2.3.1.201 | acetyl-CoA:UDP-2-acetamido-3-amino-2,3-dideoxy-alpha-D-glucuronate N-acetyltransferase |
2.7.1.227 | 1-phosphatidyl-1D-myo-inositol:a very-long-chain (2’R)-2′-hydroxy-phytoceramide phosphoinositoltransferase |
2.7.8.12 | CDP-glycerol:4-O-[(2R)-glycerophospho]-N-acetyl-beta-D-mannosaminyl-(1->4)-N-acetyl-alpha-D-glucosaminyl-diphospho-ditrans,octacis-undecaprenol glycerophosphotransferase |
2.7.8.36 | UDP-N,N’-diacetylbacillosamine:tritrans,heptacis-undecaprenyl-phosphate N,N’-diacetylbacillosamine transferase |
3.1.1.114 | methyl acetate acetohydrolase |
3.1.3.27 | phosphatidylglycerophosphate phosphohydrolase |
3.1.6.6 | choline-sulfate sulfohydrolase |
3.1.6.8 | cerebroside-3-sulfate 3-sulfohydrolase |
3.10.1.1 | N-sulfo-D-glucosamine sulfohydrolase |
3.2.1.11 | 6-alpha-D-glucan 6-glucanohydrolase |
3.2.1.152 | mannosylglycoprotein endo-beta-mannosidase |
3.2.1.197 | beta-1,2-D-mannoside mannohydrolase |
3.2.1.24 | alpha-D-mannoside mannohydrolase |
3.4.21.26 | prolyl oligopeptidase |
4.1.99.1 | L-tryptophan indole-lyase (deaminating; pyruvate-forming) |
4.2.2.20 | chondroitin-sulfate-ABC endolyase |
4.2.2.21 | chondroitin-sulfate-ABC exolyase |
4.2.2.3 | alginate beta-D-mannuronate—uronate lyase |
4.2.2.8 | heparin-sulfate lyase |
4.3.1.7 | ethanolamine ammonia-lyase (acetaldehyde-forming) |
5.1.1.20 | L-alanyl-D-glutamate epimerase |
5.1.3.11 | cellobiose 2-epimerase |
5.3.1.22 | hydroxypyruvate aldose-ketose-isomerase |
6.1.1.13 | D-alanine:poly(phosphoribitol) ligase (AMP-forming) |
One of them caught my eye, heparin-sulfate lyase, because micro-clots and “thick blood” are associated with these conditions with good results reported from the use of heparin for some patients.
HSGAGs are widely distributed on the cell surface and extracellular cell matrix of virtually every mammalian cell type and play critical role in regulating numerous functions of blood vessel wall, blood coagulation, inflammation response and cell differentiation.
Microbial heparin/heparan sulphate lyases: potential and applications [2012]
Bacteria – Citizen Science
This blew me away — we have over 150 people with PCAS and over 250 with ME giving us superior sample sizes. We have 145 bacteria deemed significant for one or the other. We had NONE that was in common. This gut punch gives two main options: denial and look for an excuse to disregard. or roll with the punch and roll with enzymes.
Bottom Line
The enzyme aspect is the strongest association between PACS and ME. The count is higher, but more important, we are dealing with p < 0.001 data making false positives unlikely. This leads to a model that there is no ‘magical list of bacteria involved’ but a diverse array of bacteria that may be different for each person — but united in the over production of enzymes. This shifts the microscope of research into a different light spectrum. This is very interesting and may require some new brain cells to be used.
Using this information to improve..
If Enzymes estimate trumps bacteria levels (in a statistical sense), then we need to look at the enzyme levels and deduce for each one of concern, which collection of bacteria needs reduction — even when they are in the normal range. It is the aggregation of bacteria where the issue arises, not individual bacteria or specific subsets of bacteria.
A few examples may illustrate things a little
Example: (S)-3-hydroxy-3-methylglutaryl-CoA acetoacetate-lyase (acetyl-CoA-forming) a.k.a. EC 4.1.3.4, which was the most significant for PACS in the post: Long COVID – an update. There are some 2000+ taxon associated with it. We look at the averages for these below.
Rank | Tax_Name | With PACS | Without PACS | TScore | DF |
species | Faecalibacterium prausnitzii | 138151 | 109604 | 3.796775 | 667 |
species | Pseudomonas viridiflava | 53 | 25 | 2.628108 | 32 |
species | Comamonas kerstersii | 125 | 40 | 2.600388 | 54 |
species | Pseudomonas aeruginosa | 62 | 31 | 1.824644 | 43 |
species | Emticicia oligotrophica | 2303 | 967 | 1.727619 | 455 |
species | Denitratisoma oestradiolicum | 42 | 24 | 1.650657 | 22 |
species | Granulicella tundricola | 29 | 21 | 1.61225 | 48 |
species | Bacillus subtilis | 40 | 19 | 1.374431 | 17 |
species | Niabella soli | 31 | 24 | 0.963943 | 16 |
species | Ralstonia insidiosa | 53 | 38 | 0.914874 | 36 |
species | Oligella ureolytica | 51 | 32 | 0.89768 | 19 |
species | Glaciecola nitratireducens | 27 | 24 | 0.67899 | 66 |
species | Bacillus halotolerans | 32 | 28 | 0.371219 | 58 |
species | Acidaminococcus intestini | 749 | 624 | 0.357674 | 146 |
species | Acinetobacter guillouiae | 67 | 63 | 0.098956 | 18 |
For another one, we see the pattern stronger. Below we see the difference of Faecalibacterium prausnitzii is around 30,000 units. Looking at the other contributors, we see an additional 40,000 units. These extra units doubles the shift (and thus significance) of the enzyme above that of a single bacteria. Some of these are deemed healthy usually, for example: Akkermansia muciniphila which was at the 78%ile for Without PACS and 83%ile with PCAS. Neither would be deem to be outliers.
Rank | Taxon Name | With PACS | Without PACS | t-score | DF |
species | Faecalibacterium prausnitzii | 138151 | 109604 | 3.796775 | 667 |
species | Sutterella wadsworthensis | 9626 | 6772 | 2.380718 | 452 |
species | Aliarcobacter skirrowii | 3756 | 21 | 2.223602 | 17 |
species | Akkermansia muciniphila | 19096 | 12290 | 1.896922 | 547 |
species | Desulfovibrio desulfuricans | 1423 | 469 | 1.769772 | 32 |
species | Emticicia oligotrophica | 2303 | 967 | 1.727619 | 455 |
species | Enterococcus casseliflavus | 1965 | 81 | 1.635666 | 38 |
species | Porphyromonas asaccharolytica | 1350 | 254 | 1.59988 | 186 |
species | Bacteroides fragilis | 8080 | 5595 | 1.523991 | 489 |
species | Bifidobacterium dentium | 1454 | 461 | 1.433823 | 239 |
species | Phocaeicola dorei | 35482 | 29075 | 1.396731 | 649 |
species | Corynebacterium aurimucosum | 1105 | 407 | 1.275234 | 96 |
species | Bacteroides eggerthii | 14379 | 10345 | 1.108857 | 263 |
species | Corynebacterium jeikeium | 1897 | 723 | 0.858282 | 70 |
species | Phocaeicola coprophilus | 6496 | 3642 | 0.856783 | 152 |
species | Desulfovibrio piger | 2032 | 1534 | 0.848976 | 141 |
species | Megamonas funiformis | 1677 | 1130 | 0.620283 | 90 |
species | Hathewaya histolytica | 2890 | 2729 | 0.467066 | 660 |
species | Haemophilus parainfluenzae | 1343 | 1250 | 0.282656 | 500 |
species | Mesoplasma entomophilum | 1182 | 1069 | 0.230055 | 294 |
species | Phocaeicola vulgatus | 51403 | 51213 | 0.034398 | 665 |
Going Forward
The logical approach is simple to describe. For a person with the symptom, determine the enzymes which are abnormal. Determine the bacteria that are too high (even if only a little). Then use the suggestions AI Engine to determine the substances that will affect the greatest number of these bacteria to shift in the desired direction without encouraging other bacteria that could contribute to these enzymes to increase.
Now, the mathematics and complexities of this computation is a different matter but well within the power of today’s computer.
Recent Comments